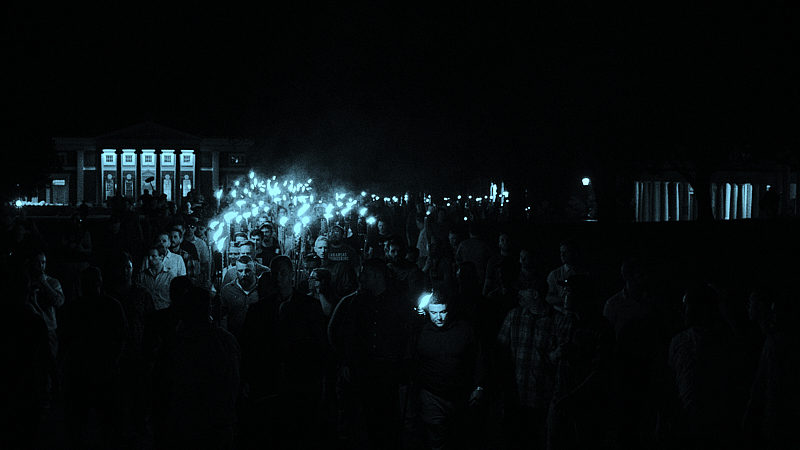
What Social Media Platforms Miss About White Supremacist Speech
Very Fine People
The Belfer Fellowship
Author
Libby Hemphill is an associate professor in the University of Michigan's School of Information and the Institute for Social Research. She studies politicians, non-profit organizations, and television fans to understand how people use social media to organize, discuss, and enact social change. She also develops automated mechanisms for moderating and classifying content in social media in order to reduce toxicity in online conversations. Dr. Hemphill received a Ph.D. and M.S. in Information from the University of Michigan and an A.B. from the University of Chicago.
Photography
Daniel Hosterman is a software developer and documentary photographer from North Carolina.
The Belfer Fellowship
The Belfer Fellowship was established by the Robert Belfer Family to support innovative research and thought-leadership on combating online hate and harassment for all. Fellows are drawn from the technologist community, academia, and public policy to push innovation, research and knowledge development around the online hate ecosystem. ADL and the Center for Technology and Society thank the Robert Belfer Family for their dedication to our work, and their leadership in establishing the Fellows program.
Center for Technology & Society
In a world riddled with cyberhate, online harassment and misuses of technology, the Center for Technology & Society (CTS) serves as a resource to tech platforms and develops proactive solutions. Launched in 2017 and headquartered in Silicon Valley, CTS aims for global impacts and applications in an increasingly borderless space.
It is a force for innovation, producing cutting- edge research to enable online civility, protect vulnerable populations, support digital citizenship and engage youth. CTS builds on ADL’s experience over more than a century building a world without hate and supplies the tools to make that a possibility both online and offline.
Executive Summary
Social media platforms provide fertile ground for white supremacist networks, enabling far-right extremists to find one another, recruit and radicalize new members, and normalize their hate. Platforms such as Facebook and Twitter use content matching and machine learning to recognize and remove prohibited speech, but to do so, they must be able to recognize white supremacist speech and agree that it should be prohibited. Critics in the press[1] and advocacy organizations[2] still argue that social media companies haven’t been aggressive or broad enough in removing prohibited content. There is little public conversation, however, about what white supremacist speech looks like and whether white supremacists adapt or moderate their speech to avoid detection.
Our team of researchers set out to better understand what constitutes English-language white supremacist speech online and how it differs from general or non-extremist speech. We also sought to determine whether and how white supremacists adapt their speech to avoid detection. We used computational methods to analyze existing sets of known white supremacist speech (text only) and compared those speech patterns to general or non-extremist samples of online speech. Prior work confirms that extremists use social media to connect and radicalize, and they use specific linguistic markers to signal their group membership.[3] We sampled data from users of the white nationalist website Stormfront and a network of “alt-right” users on Twitter. Then, we compared their posts to typical, non-extremist Reddit comments.[4]
We found that platforms often miss discussions of conspiracy theories about white genocide, Jewish power, and malicious grievances against Jews and people of color. Platforms also let decorous but defamatory speech persist. With all their resources, platforms could do better. With all their power and influence, platforms should do better.
We determined five key ways that white supremacist speech is distinguishable from commonplace speech:
- White supremacists frequently referenced racial and ethnic groups using plural noun forms (e.g., Jews, whites). Pluralizing group nouns is not in itself offensive, but in conjunction with antisemitic content or conspiracy theories, this rhetoric dehumanizes targeted groups, creates artificial distinctions, and reinforces group thinking.
- They appended “white” to otherwise unmarked terms (e.g., power). In doing so, they racialized issues that are not explicitly about race and made whiteness seem at risk. By adding “white” to so many terms, they center whiteness and themselves as white people in every conversation.
- They used less profanity than is common on social media. When white supremacists are criticized, t hey claim they are being civil and focus on others’ tone rather than their arguments. Avoiding profanity also allows them to avoid simplistic detection based on “offensive” language and to appear respectable.
- Their posts were congruent on extremist and mainstream platforms, indicating that they don’t modify their speech for general audiences or platforms. Their linguistic strategies —using plural noun forms, appending “white,” and avoiding profanity—are similar in public (Reddit and Twitter) and internal ( in-group) conversations on extremist sites (Stormfront). These consistent strategies should make white supremacist posts and language more readily identifiable.
- Their complaints and messages stayed consistent from year to year. Their particular grievances and bugaboos change, but their general refrains do not. For instance, they discuss white decline (lately in the form of “Great Replacement” theory, codified in 2011), conspiracy theories about Jews, and pro-Trump messaging. The consistency of these topics makes them readily identifiable.
- They racialized Jews; they described Jews in racial rather than religious terms. Their conversations about race and Jews overlap, but their conversations about church, religion, and Jews do not.
Given identifiable linguistic markers and consistency across platforms over time, social media companies should be able to recognize white supremacist speech and distinguish it from general, non-toxic speech. As a small team of faculty and students, we used commonly available computing resources, existing algorithms from machine learning, and dynamic topic modeling to conduct our study.
We recommend that platforms use the subtle but detectable differences of white supremacist speech to improve their automated identification methods:
- Enforce their own rules. Platforms already prohibit hateful conversations, but they need to improve the enforcement of their policies.
- Use data from extremist sites to create detection models. Platforms have used general internet speech to train their detection models, but white supremacist speech is rare enough that current models cannot find it in the vast sea of internet speech. Automated approaches should also use computational models and workflows specific to extremist speech.
- Look for specific linguistic markers (plural noun forms, whiteness). Platforms need to take specific steps when preparing (that is, preprocessing) language data to capture these differences.
- De-emphasize profanity in toxicity detection. White supremacists' lack of profanity in their online conversations challenges our conception of toxic speech. Platforms need to focus on the message rather than the words.
- Train platform moderators and algorithms to recognize that white supremacists’ conversations are dangerous and hateful. Tech companies need to take seriously threats to incite violence, attacks on other racial groups, and attempts to radicalize individuals. Remediations include removing violative content and referring incidents to relevant authorities when appropriate.
Social media platforms can enable social support, political dialogue, and productive collective action. But the companies behind them have civic responsibilities to combat abuse and prevent hateful users and groups from harming others. In this report, we detail our findings and our recommendations for how companies can fulfill those responsibilities.
[1] E.g., Andrew Marantz, “Why Facebook Can’t Fix Itself,” The New Yorker, October 9, 2020, Kari Paul, “‘It Let White Supremacists Organize’: The Toxic Legacy of Facebook’s Groups,” The Guardian, February 4, 2021, Jeff Horwitz, “Facebook Knew Calls for Violence Plagued ‘Groups’, Now Plans Overhaul,” WSJ Online, January 31, 2021, Ali Breland, “Reddit Finally Banned The_Donald. That Won’t Stop the Hate It Unleashed,” Mother Jones, accessed January 4, 2022.
[2] E.g. ADL’s, Stop Hate for Profit, accessed January 4, 2022.
[3] Anti-Defamation League, “The Extremist Medicine Cabinet: A Guide to Online ‘Pills,’” November 2019; Jessie Daniels, Cyber Racism: White Supremacy Online and the New Attack on Civil Rights (Lanham, MD: Rowman & Littlefield Publishers, 2009).
[4] Stormfront bills itself as a meeting place for White Nationalists, and users in our Twitter sample came from an audience for “alt-right” content. In this report, we use the term “white supremacist” to refer to these users collectively because they affiliate themselves with these movements. Members of these groups of users authored all of the posts we considered to be “white supremacist speech.”
Introduction
White supremacists have long recognized the internet’s potential to help them reach new audiences and rally their existing adherents. David Duke, one of America’s most recognizable white supremacists, said, “The internet gives millions access to the truth that many didn’t even know existed. Never in the history of man can powerful information travel so fast and so far. I believe that the internet will begin a chain reaction of racial enlightenment that will shake the world by the speed of its intellectual conquest.”[5] Extremists intentionally seed disinformation, hoaxes, and memes to amplify and normalize their messages.[6] White supremacists build community and widely espouse their views through both extremist and mainstream social platforms.
Some scholars, like Jessie Daniels[7] and Tarleton Gillespie,[8] argue that white supremacy thrives online when we imagine the internet (and technology broadly) as “race-less.”[9] Often, communities and platforms selectively enforce existing rules against hateful speech. Inadequate enforcement means platforms don’t do enough to prevent white supremacists’ threats. Mainstream social media platforms (such as Facebook, YouTube, and Twitter) prohibit explicit hate speech and language that encourages violence, but they have many gaps, and they appear unable or unwilling to do more to stop white supremacy, as prior ADL research shows.
Platforms use a combination of algorithms and human reviewers to make judgments about whether content violates their rules. They face many challenges, including defining what constitutes “hate speech” or a threat of violence and handling the massive scale of user-generated content.
Extremist groups know that these rules are tough to define and enforce.[10] White supremacists are adept at hiding in plain sight by using language that differs only slightly from acceptable speech (“they [Jews] should be thrown out”), masking nefarious intent with thinly veiled phrases (“preserve our culture”) and appropriating innocuous images in racist, bigoted ways (e.g., Pepe the Frog).[11] Platforms exempt humor and parody from their hate-speech policies, as Twitter states: “Users are allowed to create parody, newsfeed, commentary, and fan accounts on Twitter, provided that the accounts follow the requirements below.” (Such policies are not always made explicit, however, since some platforms detail only what they prohibit, not what they allow.) But it’s challenging to define what constitutes “humor” or “parody” in terms of what’s permissible, an ambiguity that extremists exploit. In order to understand whether a particular comment is satirical, one needs to understand its context. Many automated content-moderation decisions are made without that context, and they risk both over-policing[12] and under-addressing[13] hate speech. But the context of a post matters as much as its content.
The context surrounding an individual post, including who posted it and which community they posted it in, helps to reveal the subtleties of problematic language and its social meaning. Algorithms can detect some egregious or overt hate speech because recognizing it doesn’t require much context. But current algorithms and methods often overlook harmful narratives and framings that do not rely on obvious slurs or known euphemisms—the exact language and tactics that white supremacists deliberately use to both engage new audiences and skirt platform rules.
The sheer volume of social media content also presents a challenge for platforms. Users post so much content to mainstream social media platforms that it’s not practicable for humans to review it all. Platforms use AI and machine learning systems to help reduce the volume of content to review. Computer algorithms are only as good as their programming (that is, the rules and processes we teach them), however, so developers need to understand the distinctions we want algorithms to make. Hateful content can cause extensive harm to targeted groups, but it is often hard to detect automatically, given its low prevalence. Even a few instances of hate speech or incitement to violence can have disproportionate negative effects in two key ways. First, hateful content directly harms those targeted.[14] Second, because social media platforms amplify and spread information, such postings and comments can rack up millions of views and reach users who are susceptible to radicalization and recruitment efforts.
Because of limitations in identifying white supremacist speech on mainstream platforms, we used content from Stormfront, a popular and notorious white supremacist website, to seed our models. We compared this dataset to content by a network of “alt-right” sympathizers on Twitter, a subset of white supremacists who use the term “alt-right” to rebrand white supremacy. Together, the content from Stromfront and from the dataset of self-identified “alt-right” users on Twitter serve as our samples of white supremacist speech, although they may not represent the same actual users. We then compared these datasets with non-white-supremacist samples from Reddit. To do so, we sampled comments from /r/all, a compendium of popular posts across a portion of Reddit, to serve as examples of typical, non-white-supremacist internet speech (“mainstream speech”).
With these three datasets, we compared textual content from Stormfront, alt-right Twitter users, and general Reddit users by using text similarity and topic modeling techniques. These two computational tools help to identify properties of texts that distinguish explicitly white supremacist from general or non-white-supremacist content, to group content into topics, and to detect new terms and phrases within those topics. Unlike keyword searches, our methods identify differences between speech patterns among these different communities online.
We were able to collect and analyze millions of posts from three different platforms using computing platforms and power available to consumers. All of the computational techniques we used are open source, meaning that anyone can read and use the code as we did.[15] We hosted all our computing applications on servers available on commercial cloud services through Amazon Web Services or to researchers at the University of Michigan through our Advanced Research Computing office. While the servers we used are more powerful than those in most current desktop or laptop computers, they were not very powerful compared to the resources that computer scientists and social media platforms normally use. For instance, when we generated topic models, one of the more computationally demanding steps, we used only four CPUs and 24GB of RAM. Platforms should be able to do better with all their human and computing resources.
Social media platforms have become vital spaces for public discourse and participation in social life. We all should be able to use and benefit from social media, but the presence of white supremacy online harms marginalized people and drives them from digital public spaces. Tech companies have the power to make online spaces inclusive for marginalized users, increasing social participation rather than enabling far-right recruitment and radicalization.
[5] Daniels, Cyber Racism: White Supremacy Online and the New Attack on Civil Rights, 3.
[6] Alice Marwick and Rebecca Lewis, “Media Manipulation and Disinformation Online” (Data & Society, May 15, 2017), https://datasociety.net/wp-content/uploads/2017/05/DataAndSociety_MediaManipulationAndDisinformationOnline-1.pdf.
[7] Jessie Daniels, “The Algorithmic Rise of the ‘Alt-Right,’” Contexts 17, no. 1 (February 1, 2018): 60–65, https://doi.org/10.1177/1536504218766547; André Brock, “Beyond the Pale: The Blackbird Web Browser’s Critical Reception,” New Media & Society 13, no. 7 (November 1, 2011): 1085–1103, https://doi.org/10.1177/1461444810397031; Ariadna Matamoros-Fernández, “Platformed Racism: The Mediation and Circulation of an Australian Race-Based Controversy on Twitter, Facebook and YouTube,” Information, Communication and Society 20, no. 6 (June 3, 2017): 930–46, https://doi.org/10.1080/1369118X.2017.1293130; Eugenia Siapera and Paloma Viejo-Otero, “Governing Hate: Facebook and Digital Racism,” Television & New Media 22, no. 2 (February 1, 2021): 112–30, https://doi.org/10.1177/1527476420982232.
[8] Tarleton Gillespie, “The Politics of ‘platforms,’” New Media & Society 12, no. 3 (2010): 347–64, http://nms.sagepub.com/content/12/3/347.short.
[9] Lisa Nakamura, Cybertypes: Race, Ethnicity, and Identity on the Internet (Florence, UK: Routledge, 2013), 85.
[10] Daniels, Cyber Racism: White Supremacy Online and the New Attack on Civil Rights.
[11] Daniels, “The Algorithmic Rise of the ‘Alt-Right.’”
[12] Sophia Cope, Jillian C. York, and Jeremy Gillula, “Industry Efforts to Censor Pro-Terrorism Online Content Pose Risks to Free Speech,” Electronic Frontier Foundation, July 12, 2017, https://perma.cc/NUM5-Q9HY.
[13] Richard Ashby Wilson and Molly K. Land, “Hate Speech on Social Media: Content Moderation in Context,” Connecticut Law Review 52, no. 3 (2021): 1029–76, https://papers.ssrn.com/abstract=3690616.
[14] Francesca Stevens, Jason R. C. Nurse, and Budi Arief, “Cyber Stalking, Cyber Harassment, and Adult Mental Health: A Systematic Review,” Cyberpsychology, Behavior and Social Networking 24, no. 6 (June 2021): 367–76, https://doi.org/10.1089/cyber.2020.0253; Maeve Duggan, “Online Harassment 2017,” Pew Research Center, 2017.
[15] Data and code specific to this project are available through openICPSR at http://doi.org/10.3886/E156161V1.
Methodology
Understanding the differences between white supremacist and non-white-supremacist speech allows for the design of algorithms that can better detect harmful content, recognizing adaptations that extremists use to circumvent keyword detection and being updated as needed. In order to understand white supremacist language online, we needed a large sample of it to analyze. We also needed data from mainstream users to serve as a baseline for comparison. Because white supremacists might use different language when talking to each other in their own spaces than they use in shared spaces, we needed data from both extremist and mainstream platforms. The prominent French sociologist Pierre Bourdieu[16] explains that social relationships drive language choices, and people reinforce and reproduce those relationships when they adopt in-group language. For white supremacists, sharing content online signals belonging and reinforces the norms of the group.[17]
To get data from white supremacists talking to each other, our team downloaded nearly 275,000 posts from the white nationalist discussion board Stormfront. Stormfront users call themselves “White Nationalists” and claim to be “the voice of the new, embattled White minority!”[18] We used a similar approach to identifying useful data from Twitter. We drew on VOX-Pol’s Alt-Right Twitter Census[19] to identify extreme right-wing users on Twitter as a sample of extremists on a mainstream platform; we used data from 2,237 Twitter accounts. This “alt-right” Twitter census was created by identifying participants in a network of users who affiliated themselves with the alt-right, a term that members use to rebrand white supremacy.[20] The alt-right users on Twitter may or may not overlap with Stormfront users, something this study did not investigate.[21] Together, the Stormfront users’ comments and Twitter alt-right users’ posts serve as our “white supremacist speech” sample. White supremacists believe that white people should have dominance over people from other backgrounds and that white culture is superior to others.[22]
To get data from mainstream users, we sampled Reddit, one of the most popular social media sites. We sampled comments from /r/all, an aggregated feed of popular posts from across Reddit’s communities, to serve as examples of typical internet speech.
All together, the data we analyzed include extremists on an extremist platform, Stormfront, extremists in an alt-right network on Twitter, and general users on Reddit. We pulled posts made between 2015 and 2021, including 274,668 from Stormfront, 755,807 from Twitter, and 509,982 from Reddit (see Table 1).
Limitations based on our sample
Our two samples of extremists, Stormfront users and alt-right users on Twitter, allow us to ensure that our samples include white supremacist speech. Users in these groups may or may not overlap and may not all identify the same way. We consider Stormfront users to be extremists and white supremacists because the platform is explicitly a site for white supremacy. The alt-right Twitter census uses indicators such as positive mentions of the term “alt-right” and participation in alt-right networks to identify alt-right Twitter accounts. Because this term is used by adherents to make extremist white supremacy more acceptable, ADL considers identification with the “alt-right” to be a signifier of white supremacy.
Our extremist samples do not represent all extremist speech or even every way in which these individuals communicate. These users may change their language, or code-switch, between platforms and discussions. Other users we didn’t study may also identify as white supremacists. For instance, because of the method we used to identify candidate accounts on Twitter, our Twitter data may contain people who do not identify themselves as “white supremacist” even though they follow accounts that do. We also cannot tell whether Stormfront users and Twitter users in our sample are the same individuals using different platforms. We did not compare usernames or statistics, nor did we investigate individual users’ comments in this study.
To ensure our samples were not simply representative of conservative or right-wing speech, we compared users on the white supremacist forum Stormfront and on alt-right Twitter to conservative politicians, based on data from prior research.[23] When we looked at the actual words that are similar, "white" appeared in the top three unique words for both Stormfront and the alt-right network on Twitter. Among the politicians, however, speech was more mundane: their top three unique words were “house,” “today,” and “great.” Politicians, of course, may curate their speech differently than other users do, but the frequent use of “white” as a modifier is a pattern that distinguishes far-right users on both Stormfront and alt-right Twitter.
Colloquial conversations, like those we studied on Reddit, also exhibit linguistic variation and may contain both overt and coded white supremacist speech. Because we relied on users’ self-identification and participation in extremist networks, we do not have labels for comments posted to Reddit (we had no comparable information about Reddit users’ identities or affiliations). Future work should more closely examine strategies white supremacists use to avoid detection or to shift innocuous conversations toward more hateful exchanges.
Our sample does not include posts made but then deleted by either users or platforms. We cannot know what content was removed or why, because the platforms do not share this information. We are only able to comment on what platforms, especially Twitter, miss about white supremacist speech, because these tweets and the users who posted them were not removed shortly after they were posted. Some of the users and posts in our sample may have been deleted, banned, or suspended since we collected their data; it’s possible that Twitter or Reddit removed posts or users more recently.
To analyze the data, we used existing techniques from natural language processing (NLP) and machine learning (ML). By natural language processing, we mean computational approaches to representing and studying written language. By machine learning, we mean training algorithms to assign content to various classes by iteratively incorporating feedback on their performance. These algorithms can both identify and learn linguistic patterns. Together, NLP and ML help us to study texts at a scale and speed that isn’t possible for humans alone and identify patterns that are rare or subtle, which humans might miss. Instead of creating new algorithms or mathematical techniques, we used common computational approaches for measuring the frequency and importance of words and phrases within documents, comparing documents to each other, and categorizing or grouping documents based on attributes they share. In the process, we also manually read posts from all of these platforms, and we used various graphs and figures to understand and explore the data. The computational techniques we used—text similarity comparisons, topic modeling, and word embeddings—let us look at thousands of posts and uncover similarities and differences that wouldn't be apparent if we were reading them individually.
These computational techniques allow us to examine complete sets of documents like “all of Stormfront” rather than samples of text that are returned by keyword searches or other ways of sampling from documents. We also use NLP and ML to discover key terms from texts rather than specifying the terms a priori. This allows us to identify novel patterns and terms that were not already known.
Topic modeling, for example, is a data mining technique that identifies semantic similarities between documents. It then clusters documents into groups based on these similarities, and these document groups are called “topics.” “Topics” are essentially algorithmically created collections of documents in which certain words and/or phrases appear more or less frequently than they do in other documents, without a priori knowledge of those words or phrases. Topic modeling algorithms assign numbers to these document groups and indicate which phrases or words are unique to each group. Then, human analysts give recognizable names to the document groups that topic modeling identifies.
For example, one topic our model discovered was indicated by the presence of the words “time,” “China,” “media,” and “virus.” Documents in this group appeared in 2020. We labeled this group “coronavirus.” We used topic modeling to get a sense of the general semantics within documents. Because topic models are a general tool, they are less accurate than supervised learning methods that use human input to cluster documents manually or keyword searches that look for specific words. On their own, topic models cannot distinguish euphemisms or specific white supremacist phrases; they must be reviewed and analyzed manually to identify and name the themes they surface, if any.
Other topics we identified on Stromfront through topic modeling in our coding schema included Germany (including references to Hitler); white genocide; Jews; the Hungarian Empire; years of significant events; American-Russian relations; militaries, police, and policing; President Obama, President Trump, and Vladimir Putin; YouTube; discussions about Stormfront and how it works; media and religion; and women and family. We identified 20 coherent topics and then collapsed them into three main categories: whiteness and white supremacy, politics and government, and culture.
We complemented topic models with word embeddings, a natural language processing technique that first learns how words are associated with one another based on where in documents they appear and then represents the words in a multi-dimensional vector space. Words with similar meanings have similar embeddings or representations. For instance, alternate spellings like “gray” and “grey” will have nearly identical representations, and synonyms like “small” and “little” will have similar representations. We used an unsupervised word-embedding approach where we passed all the text from a set of documents through an algorithm, which generated representations of words based on their similarity within those documents. We then compared embeddings of the same word—like “Black”—between two sets of documents such as “Black on Reddit” versus “Black on Stormfront.” These comparisons showed us the specific semantic space of individual words within documents; embeddings allow for more specific examination and comparison than do topic models. Together, topic modeling and word embeddings let us examine the general patterns in a set of documents and to compare the use of specific words in those documents.
Sidebar: What Is Machine Learning?
Machine learning refers to processes where algorithms take some rules we can explain and learn how to apply them to new content. In our case, we provided algorithms training data about what content was white supremacist or not, and the algorithms learned to categorize content we weren’t sure about. Computers can't understand content directly; we have to build representations of the content that computers and algorithms can understand.
Word embeddings
One way to represent content is through something called word embeddings. A word embedding is a vector representation of a word that can have more dimensions than humans can visualize or comprehend.
We often use embeddings to understand how similar words and phrases are to one another. One way to see the differences between white supremacist use of words and mainstream use of words is to build embeddings or representations of those words based on either white supremacist content or mainstream content. Then we can compare both embeddings to see how similar words we’re interested in (e.g., “Black”) are to other words in that corpus. We then compare the lists of similar words from one corpus to another to see how differently the word we’re interested in gets used in those different contexts.
We trained word embeddings using gensim’s word2vec models[24] for both Stormfront and Reddit datasets to compare words and their contexts between the two platforms.
[16] Pierre Bourdieu, Language and Symbolic Power (Cambridge, MA, USA: Harvard University Press, 1991).
[17] Julia R. DeCook, “Memes and Symbolic Violence: #proudboys and the Use of Memes for Propaganda and the Construction of Collective Identity,” Learning, Media and Technology 43, no. 4 (October 2, 2018): 485–504, https://doi.org/10.1080/17439884.2018.1544149.
[18] https://www.stormfront.org/forum/. Retrieved October 9, 2021.
[19] J. M. Berger, “The Alt-Right Twitter Census: Defining and Describing the Audience for Alt-Right Content on Twitter” (VOX-Pol Network of Excellence, 2018).
[20] “Alt Right: A Primer on the New White Supremacy” (Anti-Defamation League), accessed October 20, 2021.
[21] According to J. M. Berger, the census’s author, they created the dataset by manually identifying 439 “seed accounts” that “self-identified with some spelling or punctuation variation of ‘alt-right’ in the account’s username, display name or the Twitter bio field” and then identified 5,000 of their followers. The census describes the alt-right as more of an “extremist political bloc” than a “fully formed extremist ideology” and notes that the key themes dominating these accounts were “support for US President Donald Trump, support for white nationalism, opposition to immigration (often framed in anti-Muslim terms), and accounts primarily devoted to transgressive trolling and harassment.”
[22] “White Supremacy" (Anti-Defamation League), accessed January 4, 2022.
[23] Libby Hemphill, Annelise Russell, and Angela M. Schöpke-Gonzalez, “What Drives U.S. Congressional Members’ Policy Attention on Twitter?,” Policy & Internet, 2020, https://doi.org/10.1002/poi3.245; Libby Hemphill and Angela M. Schöpke-Gonzalez, “Two Computational Models for Analyzing Political Attention in Social Media,” Proceedings of the AAAI Conference on Web and Social Media 14, no. 1 (2020): 260–71.
[24] Radim Řehůřek and Petr Sojka, “Software Framework for Topic Modelling with Large Corpora,” in Proceedings of the LREC 2010 Workshop on New Challenges for NLP Frameworks (Valletta, Malta: ELRA, 2010), 45–50.
Results
General Patterns in White Supremacist Speech Online: Whiteness, Politics, and Culture
Our findings show that, on Stormfront and Twitter, white supremacists talk about whiteness, politics (especially the U.S. president), women, media, and specific public policies. White supremacists also talk about race explicitly and often. For example, their conversations frequently reference their white identity; they mention “white decline,” “white people,” or “whites.” Virtually no other users use the term “white” in these ways. General or mainstream users more often talk about entertainment, such as online games and movies. These patterns among white supremacists are consistent across platforms and from year to year. They distinguish themselves from non-white-supremacists through their word choice, syntax, and topics of interest.
Stormfront: When white supremacists talk to each other on an extremist network
Words and syntax. First, we examined the individual words and phrases that appeared on Stormfront. The words “white” or “whites” appear in 19 percent of posts on Stormfront. Altogether, “Jew” or “Jews” and “Black” or “Blacks” appear in roughly 7 percent of posts. “Negro” or “negroes” appear in another 2 percent of posts. Other derogatory terms for Black people and Jews (e.g., the n-word, heeb, yid) appear in only a handful of posts. Table 2 shows the rates of these and other words per 100,000 posts on both Stormfront and Reddit.
What makes these white supremacists distinct from mainstream speakers is that they explicitly and frequently discuss racial and ethnic groups. Stormfront users talk about other racial groups explicitly, often mentioning “Blacks” or “Asians.” Notably, they write about Jews and Jewish people more when they talk about race rather than religion. Although they talked about church and family, those conversations usually didn’t overlap with discussions of Jews. Conversations about Black people, conspiracies, or power, however, overlapped regularly with conversations about Jews. These overlaps imply that white supremacists see Jews as different from “white” and other races.
Recent research suggests that racist actors use coded language to evade detection by content-moderation algorithms.[25] Computer scientists Rijul Magu and Jiebo Luo found that users substituted common, inoffensive words for references to social groups; for instance, “Google” for “Black” or “Skittle” for “Muslim.” We found, however, that white supremacist and antisemitic users did not attempt to veil their references to social and ethnic groups. Or, if they use coded language, those codes are not common enough to distinguish them from benign references to Google, Skype, etc. The words most similar to “Skype” were “login” and “please send.” The word “Skittle” didn’t appear on Stormfront at all. This may be because white supremacists don’t need to use coded language when they talk to each other on Stormfront. It also may suggest that they are not using Stormfront to discuss euphemisms used elsewhere; i.e., we didn’t see instances of Stormfront users talking about how they need to say “Skittle” when they’re on Twitter.
Topics of conversations. We used dynamic topic modeling to look at the general content of the posts and compare them over time. Topic modeling, generally, is a computational approach to identifying clusters of documents that share a common theme that isn’t explicit. Dynamic topic modeling allows us to study the evolution of these implicit (topic modelers call them “latent”) themes over time by connecting topics at one time to topics at another. One element that stood out about all topics: Stormfront users foregrounded whiteness in nearly all topics whether they were discussing cultural, religious, political, or social issues. Below is an excerpt from a Stormfront comment on a general graphics/artwork forum. The author discussed whether to include “white” or “European” in a new flag graphic for use on the Stormfront site:
When you hear someone saying "i am white", then he/her is totaly defined. When you hear someone saying "i am european", today he/her can be black/asian/arab... Why? Ask yourself why blacks are calling themselfs "german", "dutch", "french" etc. You can use word "european" to be politicaly correct, or to atract much more ordinary people to it, but if you wanna truly define your origins, use the word "white".
We classified this comment as “white supremacy” rather than “culture,” even though it was in a forum about artwork, because it refers to races, countries of origin, and other identity categories.
Some documents received nearly identical probabilities for multiple topics, indicating that there may be overlap between topics like “domestic policy” and “policing.” We collapsed topics into three primary categories for ready comparison and minimal overlap: politics, culture, and white supremacy. We placed both “domestic policy” and “policing” in the broader “politics” category; we included other policy discussions and references to political debates in that category as well. Conversations where Stormfront users discussed women and families, the media, and religion, we labeled “culture” because of their co-occurrence with one another and not with “Jew,” “Muslim,” or other religious groups. Some terms suggest overlap between categories. For instance, both “Pierce” and “William” appear in “culture” because they were related to a discussion of William Pierce’s book. However, William Pierce was an infamous neo-Nazi, so discussions of his books are also related to white supremacy. We included explicit discussions of whiteness, racism, Jews, and 20th-century white supremacist movements in Germany and Hungary in “white supremacy.”
Our models indicate that documents containing the word “Jew” or “Jews” are more similar to those that contain “white” and “race” than they are to those that contain other words about religion (e.g., “Christianity” or “biblical”). For that reason, we have included topics that contain the word “Jew” with other white supremacist topics instead of with those about religion. This finding parallels how white supremacists understand Jews in racial rather than religious terms, as we detail in the following section.
Table 4 shows the distributions of these topics from 2016 to 2020. The volume of posts in the white supremacy category stayed relatively stable throughout the five-year period, accounting for nearly 25 percent of all posts. The graph indicates that an increase in political discussions mirrored a decline in conversations about cultural topics. Much of the increase in the politics topic came from discussions about the U.S. and Russia. A decrease in posts about media and religion drove the reduction in culture conversations during that same period.
The U.S. presidency was a common topic throughout the period. The tenor of those conversations was different, essentially consisting of racialized comments about Obama and pro-Trump messages, but the overall level of attention to the president was similar throughout (5–7 percent of posts).
What was missing from their conversations? Stormfront users did not discuss domestic policies around education, housing, or poverty in the data available to us. The only domestic issues that received measurable attention were immigration and policing, as our topic modeling shows.
Twitter: When white supremacists participate in public conversations on a mainstream platform
Words and syntax. Like Stormfront users, extremists on Twitter often used plural noun forms to refer to racial groups, mentioned these groups often, and used “white” as an adjective for non-racialized nouns. The example tweet thread in Figure 5 illustrates how white supremacists view Jews in racial terms. The original tweet doesn’t mention Jews explicitly; instead, it draws parallels between racism and conservatism. Whether those parallels are in jest or sincere isn’t clear from the tweet alone. In the tweets that follow, however, we see another user try to call out white privilege, and then two more users employ white supremacist terms. The first white supremacist response accuses the responding user of being “an anti-White hate monger.” The second marks “Jews” as distinct from “whites” and complains about perceived bias in media coverage.
White supremacists’ user names or handles on Twitter also set them apart from other users. Sometimes, the user names contained hateful slurs or white supremacist references even when the account’s posts seemed innocuous. Examples include “gaston_chambers” and “futureusrefugee.” The individual words within the name are not harmful on their own, but the names reference the Holocaust and U.S. immigration policy in hateful ways. Extremists have been using their handles to promote racism and make themselves visible for years.[26] This move is not new or limited to the users in our sample.
Topics of conversation. The same three general topics (politics, culture, and white supremacy) appeared among extremists on Twitter. The distributions of these topics on Twitter, however, were different. As the graph in Figure 6 shows, they remained consistently distributed over time. The Twitter users we studied talked about white supremacy or used antisemitic language more often than the Stormfront users did. Users on both platforms also talked about politics and culture; the shift toward politics and away from culture that we observed on Stormfront did not appear on Twitter. We cannot speculate on these differences based on the data analyzed here, but prior research shows that extremist groups use Twitter and other mainstream social media sites to recruit and radicalize affiliates.[27] Instead, the Twitter users were relatively consistent in the attention they paid to these topics over the period we studied.
Discussions we categorized as white supremacy (based on the terms that appeared) were common among the Twitter users in our sample (see Figure 6). Tweets in this category included uses of racial and ethnic slurs. For instance, the tweet in Figure 7 refers to COVID-19 as “wuflu,” which is shorthand for “Wuhan Flu,” a xenophobic name for the virus. Tweets in this category also included phrases such as “white people,” “white women,” “whites in America,” “a white minority,” “good old days,” and “Americans deserve better.”
Within the white supremacist topics, discussions of Jews and the perceived threats they pose to white people were common and distinct.[28] Conspiracy theories about Jewish power in media and politics were also common in these topics. In the tweet below (Figure 8), “Christians for Truth” posted a headline from a story on its site claiming that Jews maintain a media monopoly. Claims like this are not new, and they recur in this dataset. The specific people mentioned (in this case, a rabbi in Moscow) change, but the claim of a Jewish media monopoly repeats.[29]
Many of the politics topics were about the U.S. president, because extremist users frequently mention the president. This was true of President Obama and President Trump, who were each in office during the period we examined. Almost 20 percent of all tweets mentioned Trump or his presidency; this pattern began during the 2016 elections. Tweets that mentioned President Trump often discussed immigration and travel policies. For instance, white supremacist leader Richard Spencer tweeted to ask the president to deport Jose Antonio Vargas, an undocumented immigrant and activist:
Spencer’s tweet also illustrates the overlapping patterns of white supremacist speech on Twitter (i.e., in public conversations) and on Stormfront (i.e., among white supremacists); he calls Vargas an “anti-white activist.” His reference to immigration policy is implicit, but his white supremacy is explicit.
The topic of women and media also includes common white supremacist themes. For instance, we placed the tweet by Twitter user “Bum Farto” in the “white women” topic (see Figure 10). The tweet also illustrates how white supremacists use adjective-noun phrases such as “white race” and “white women.” Bum Farto’s tweet assigns a community role to white women: specifically, that they hold a race together. Comments that emphasize women’s mothering and nurturing roles are common among white supremacists;[30] they reinforce the idea that white women are essential actors in reproducing and maintaining racially “pure” communities, an idea that undergirds white supremacist misogyny.[31]
Unique Properties of White Supremacist Speech Online
The previous section described the patterns of speech among extremists on Stormfront and Twitter. Here, we turn to the attributes of those patterns that distinguish white supremacists’ speech from other users’. Our statistical analysis shows that, compared to users on Reddit,[32] white supremacists use “white” as an adjective in more phrases, use plural noun forms to talk about racial and ethnic groups, frequently discuss race explicitly, and rarely use profanity. They are also consistent in their speech across platforms and their complaints over time. Their conversations reveal strategies for effectively communicating online: foregrounding whiteness, appearing polite, and staying on message.
White supremacists frequently reference racial and ethnic groups using plural noun forms (e.g., Jews, whites).
On each platform we analyzed, white supremacists typically used a plural noun form to talk about members of racial and ethnic groups. For instance, they used words such as “whites,” “Blacks,” and “Jews” to talk about particular groups of people. In contrast, mainstream users wrote adjective-noun phrases such as “white people” or “Black people” to refer to racial groups.
Plural noun forms are not always indicative of white supremacy, of course—referring to Jews in the plural is not inherently offensive. In some cases, like saying “Blacks,” the presence of the plural noun on its own was unique to white supremacists. In the case of “Jews,” the plural noun and another term or phrase made it recognizably white supremacist. For instance, one Stormfront comment combined “Jews” with related terms “Zionism” and “gentile”: “Zionism is a code word conceived by the jews [sic] and promoted by the jews and their gentile stooges.” In another example, a user equated Jews with characters in an online video game who experienced slavery and overthrew their oppressors using alchemy: “The goblins in WoW are clearly the jews.”[33] In this example, “Jews” is not a problem on its own, but when it appears with “goblins … are,” dehumanization is clear. Our method of comparing texts using their word embeddings showed that white supremacists are much more likely to use “Jews” in combination with hateful remarks. It is also likely that white supremacists typically refer to "the Jews" or "the Blacks," but because we removed these common articles (called "stop words" in NLP), we were not able to distinguish such instances.
Mainstream speakers don’t often mention groups like Jews, Black people, or white people. White supremacists are more likely to explicitly mention race or ethnicity within their topics of conversation or regarding people they’re either talking to or criticizing. For instance, “Jews” appears in 1/20 of Stormfront posts and just 1/10,000 of Reddit posts. So, while “Jews” may be used inoffensively, Stormfront users are much more likely to use it, and their uses were not typically benign, as our manual review confirmed. On Stormfront, it's rare for a user not to mark the racial and ethnic identity of the speaker or person they are discussing. Users say “the white woman” instead of “the woman,” or they say “that Jewish reporter” instead of “that reporter.” Among mainstream white users, “white” is likely the implied default and goes unnamed.
An earlier analysis of hate speech on Reddit by ADL and the D-Lab at the University of California at Berkeley found that “when you look for one kind of hate, you end up finding hate of all kinds.”[34] We also found that hate toward different groups appears together, especially hatred of Jews and Black people; this pattern was much more pronounced on Stormfront than on Reddit. However, though conspiracy theories about Jewish power in media and politics were also common within these topics, the algorithm did not find similar discussions of racial minorities.
They append “white” to otherwise unmarked terms (e.g., genocide). In doing so, they racialize issues that are not explicitly about race.
White supremacists on both mainstream and niche platforms use the adjective “white” and talk about race frequently. On Stormfront, “white” appears as an adjective to modify many nouns that are racially unmarked. For instance, “power,” “genocide,” and “decline” all appeared in adjective-noun phrases with “white” as the adjective (see Table 5). We found those word pairings to be rare among mainstream users.
Word choice alone is enough to tell Stormfront and Reddit users apart. We trained a supervised machine-learning algorithm using logistic regression. We chose a logistic-regression model because it is a discriminative classifier—meaning it is useful for telling two classes of objects apart—and its results are straightforward to interpret.[35] In a logistic-regression model, each feature of the text—in this case, a word or two-word phrase—receives a weight that indicates how strongly correlated with a category it is. In this case, the word with the highest weight (meaning it was most strongly associated with Stormfront) was “anti-white.” The model was 91 percent accurate when distinguishing Stormfront comments from mainstream Reddit comments. The example above shows that “anti-white” is a term employed among alt-right users on Twitter, too. This high accuracy tells us that words alone can tell (extremist) Stormfront and (mainstream) Reddit apart. Models often include other features such as users’ syntax or properties, but those attributes were not necessary here.
They use less profanity than is commonplace on social media.
Common features of white supremacist text, as this report shows, include the use of plural nouns to talk about minoritized groups, the presence of explicit racial or ethnic markers, and the use of “white” as an adjective for nouns that are not otherwise racialized. What doesn't appear in white supremacist text? Notably, profanity and racial slurs.
Profanity is common on Reddit. Table 6 uses a word shift graph to show what words are uniquely common on Stormfront and Reddit when we compare the two sites. Profane words appear on Reddit but not on Stormfront. The table also shows that “white” and “quote” appear much more often on Stormfront, while innocuous adverbs such as “like” and “please” are more common on Reddit. We also didn't often see racial slurs like the n-word or derogatory terms like “heeb” or “yid” on Stormfront. It is tricky to computationally distinguish an accepted in-group use of slurs (e.g., the n-word) from defamatory use unless we include the context of the word in our analysis.
White nationalists have talked publicly and explicitly about their attempts to appear respectable.[36] Using language that is civil or appropriate on the surface is one strategy for doing so. That users swear on Reddit and don’t on Stormfront poses a challenge for existing detection methods, because many of them treat vulgarity and profanity as classes of hate speech. It’s possible that Twitter already removed all the profane content that the users we studied posted. This is unlikely and suggests that white supremacist speech gets removed only when it's profane; if white supremacists execute their “stay presentable” strategy, their content doesn't get removed. We can't know what Twitter removed; we can only know what was still available, and it wasn't profane.
Stormfront talks explicitly about race, especially whiteness, and Reddit does not. The conversations that include “white,” “Black,” and “Jew” on Stormfront are very closely tied to one another—hateful discussions of one group also discuss others. On Reddit, the conversations are more dispersed; “white” and “Black” are more likely to show up in conversations about colors than about people. The words and terms most associated with Stormfront included race and separation terms such as “pro-white,” “anti-white,” “non-whites,” “our people,” and “white genocide.” Reddit’s most prominent words were profane (“shit,” “fuck,” “fucking”) or about the platform itself (“a bot,” “this subreddit”). When we use unsophisticated tools like slur-or-profanity detection to mark content as inappropriate, we miss polite but hateful white supremacist speech.
Their posts are congruent across both niche and mainstream platforms.
White supremacists intentionally choose their words to spread and normalize white supremacist ideology. On both Stormfront and Twitter, white supremacy, culture, and politics collectively describe nearly all of the topics they discuss. Groups on both platforms use “white” and other race markers to foreground race and ethnicity in their posts. They float conspiracy theories and lament threats to whiteness and white dominance.
One notable aspect of their political discussions is what they consistently lack. Stormfront users do not address domestic policies around education, housing, or poverty. The only domestic issues that receive measurable attention are immigration and policing. Their discussions of policing, particularly the relationships between police and Black Americans, predated the Black Lives Matter and “defund the police” movements. Similarly, every year of our data includes immigration discussions. Their interests in immigration and policing are not new.
Stormfront conversations are more focused on sociopolitical issues, especially race, while Reddit contains more casual conversation topics like gaming. It is possible that white supremacists are participating in casual, general conversations on Reddit, but we cannot distinguish their speech computationally. To identify white supremacists on the other platforms, we relied on individuals’ explicit membership (Stormfront) or alt-right affiliation (Twitter), but we did not have similar explicit identity markers for Reddit. In contrast to Redditors, Stormfront participants use political terms such as “American,” “president,” and “country” much more often. They share reports and articles more frequently. Redditors, on the other hand, talk more about games and entertainment. The relative frequency of terms like “games” and “character” makes this clear. The frequency of discussions about video games on Reddit may make it a target for white supremacists aiming to recruit and radicalize other users. We may be missing even more subtle white supremacy within these discussions.
Their complaints and messages are consistent over time.
We did not identify specific phrases or words that emerged and then persisted. Instead, the specific targets of white supremacists’ complaints changed, but the themes remained the same. Methods that identify these themes will therefore be more successful at identifying white supremacist content over time.
The specific content of their posts may shift over time, but their relative attention does not change dramatically (see Figures 4 and 6). For instance, foreign policy discussions specifically mention Russia and Iran throughout the 20 years of data available. China emerged in 2020 and only in discussions of the coronavirus. One recent shift worth noting is that discussions within the past few years have explicitly addressed politics more often and white supremacy less. One reading of that shift is that discussions of white supremacy are declining. However, prior research has shown that white supremacists’ politics are becoming mainstream,[37] and there may be more overlap between “white supremacy” and “politics” in more recent years. Figures 4 and 6 show how similar is the distribution of topics on both Stormfront and Twitter.
[25] Rijul Magu and Jiebo Luo, “Determining Code Words in Euphemistic Hate Speech Using Word Embedding Networks,” Proceedings of the 2nd Workshop on Abusive, 2018, https://www.aclweb.org/anthology/W18-5112.pdf; Rijul Magu, Kshitij Joshi, and Jiebo Luo, “Detecting the Hate Code on Social Media,” in Eleventh International AAAI Conference on Web and Social Media, 2017.
[26] Pete Simi and Robert Futrell, American Swastika: Inside the White Power Movement’s Hidden Spaces of Hate (Lanham, MD: Rowman & Littlefield Publishers, 2015), 92, http://ebookcentral.proquest.com/lib/umichigan/detail.action?docID=2081815.
[27] DeCook, “Memes and Symbolic Violence: #proudboys and the Use of Memes for Propaganda and the Construction of Collective Identity”; Carolyn Gallaher, “Mainstreaming White Supremacy: A Twitter Analysis of the American ‘Alt-Right,’” Gender, Place and Culture: A Journal of Feminist Geography 28, no. 2 (February 1, 2021): 224–52, https://doi.org/10.1080/0966369X.2019.1710472; Miriam Fernandez, Moizzah Asif, and Harith Alani, “Understanding the Roots of Radicalisation on Twitter,” in Proceedings of the 10th ACM Conference on Web Science, WebSci ’18 (New York, NY, USA: Association for Computing Machinery, 2018), 1–10, https://doi.org/10.1145/3201064.3201082.
[28] Topic models are not precise enough to support statistical comparisons, but they are helpful for seeing the general trends we present in the graphs above. When we say topics are “common and distinct,” we mean that, compared to other possible latent topics, they are readily recognizable by a naïve algorithm and that it can also distinguish between them.
[29] See the ADL’s Antisemitism Uncovered: A Guide to Old Myths in a New Era for more details about common tropes and templates levied against Jews.
[30] Alexandra Minna Stern, Proud Boys and the White Ethnostate: How the Alt-Right Is Warping the American Imagination (Beacon Press, 2019).
[31] Jennifer Fluri and Lorraine Dowler, “House Bound: Women’s Agency in White Separatist Movements,” in Spaces of Hate: Geographies of Discrimination and Intolerance in the U. S. A., ed. Colin Flint (London, UK: Taylor & Francis Group, 2003), 69–85.
[32] https://www.reddit.com/. Reddit is one of the largest, most active social media sites online. It is organized into discussion communities called “subreddits” based on the topics or users within a particular subreddit. For instance, /r/Sneakers is a subreddit dedicated to discussions of sneakers, and /r/chicago is a subreddit for discussions about the city of Chicago. While it still appears, white supremacy is less prevalent on Reddit than it is among the self-described extremists we studied on Stormfront and Twitter, and so, we chose it as our “mainstream” platform for comparison.
[33] “Goblin - WoW,” accessed January 4, 2022, https://worldofwarcraft.com/en-us/game/races/goblin.
[34] “The Online Hate Index: Innovation Brief” (Anti-Defamation League, January 2018).
[35] James H. Martin and Dan Jurafsky, Speech and Language Processing: An Introduction to Natural Language Processing, Computational Linguistics, and Speech Recognition (Upper Saddle River, N.J.: Prentice Hall, 2000).
[36] Daniels, “The Algorithmic Rise of the ‘Alt-Right.’”
[37] Gallaher, “Mainstreaming White Supremacy: A Twitter Analysis of the American ‘Alt-Right’”; Mary Bucholtz, “The Public Life of White Affects,” Journal of Sociolinguistics 23, no. 5 (November 2019): 485–504, https://doi.org/10.1111/josl.12392; Burton Speakman and Marcus Funk, “News, Nationalism, and Hegemony: The Formation of Consistent Issue Framing Throughout the U.S. Political Right,” Mass Communication and Society 23, no. 5 (September 2, 2020): 656–81, https://doi.org/10.1080/15205436.2020.1764973.
Conclusions
Platforms’ content-moderation shortcomings
We were able to distinguish white supremacist speech from general speech using techniques that are available to consumers and researchers. Platforms are not leveraging all available knowledge and resources to address white supremacy in their content-moderation systems. Current systems rely on computational methods for analyzing the language and networks of extremists online. Nearly all researchers in this area have studied hate speech generally but not white supremacist speech specifically. This lack of expertise means their detection systems are less accurate. Broadly, the two common approaches for identifying hate speech leverage dictionaries or machine learning but don’t generally incorporate sufficient capabilities to identify white supremacist content.
Dictionary approaches locate known words and phrases within texts. Many dictionary studies rely on data from Hatebase,[38] a multilingual, hierarchical dictionary of terms. Dictionary approaches require analysts to know in advance what terms or phrases to look for, and that means they are not useful for finding novel speech. Often, they leverage other features of the text (such as the syntactical relationships between words or the prevalence of similar words) to disambiguate benign uses of words in the dictionaries (e.g., “curiosity is a bitch”) from malicious uses (e.g., “He didnt call him the N word, quit being a bitch”). Because words that white supremacists use often, such as “white,” “Black,” and “Jew,” are also common and important in other communication, we can’t rely on dictionaries that mark them as unacceptable.
Supervised machine learning is another popular computational approach for detecting hate speech.[39] In supervised learning, relatively small sets of human-annotated data are used to train computational models to label data automatically. Training data indicate whether particular categories of speech (like “white supremacist”) are present or absent in a document. Often, the labels for the small set of training data are provided by crowdsourced workers on sites like Amazon Turk and Crowdflower. These workers are shown a comment and asked whether it contains various types of hate speech. The labeled data is then used to train models that also leverage information such as the syntax of the sentence and various characteristics of the users who posted the content to predict whether or not a post contains hate speech. Most crowdsourced workers do not have special expertise in identifying hate speech. Domain experts, such as anti-racism activists, provide more reliable labels,[40] but they are harder to find and employ.
Hate speech is rare relative to all speech in social media, and that makes it difficult to generate datasets large enough to train automated classifiers. Relatively few users also account for most hate speech, and training classifiers also requires data from many users so that the classifiers learn to label the speech and not just to recognize users.[41] It is expensive to generate and manually label datasets that are large and diverse enough to address those issues.
None of these computational methods have focused on white supremacist speech specifically until now. Not all white supremacist speech is hateful,[42] and not all hateful speech is white supremacist. Studying white supremacist speech presents similar challenges to studying hate speech in general: We don’t yet know all the ways in which white supremacists speak. Relative to speech generally, white supremacist speech is also very rare. Generating manual labels for white supremacist speech using current methods would be incredibly expensive.
By relying on these methods (dictionaries and existing machine-learning approaches), platforms currently fail to address white supremacy in three key ways: missing polite but toxic speech, failing to adapt data-preparation steps in natural language processing to the specifics of white supremacy, and relying on overly general language models.
Platforms miss polite but toxic speech
Many existing detection methods treat vulgarity and profanity as classes of hate speech. But our analysis shows that users on Reddit swear more, while white supremacists on Twitter and Stormfront do not. General toxicity algorithms, such as Perspective API, over-identify profane speech, while “polite” white supremacist speech goes unnoticed. Recent research highlighted differences between “swearing for emphasis” and “swearing to offend;”[43] training models to distinguish these uses of profanity will be important future work.
Often, detection algorithms leverage a dictionary like Hatebase[44] to seed their detection processes. Hatebase is a database of hateful terms from many languages, and it serves as a starting point for many popular toxicity-detection algorithms.[45] Perspective API, one of the most popular toxicity-detection tools, offers a specific profanity measure and uses its presence to determine the toxicity score of a document.[46] Extremists have realized that when they are more presentable, they gain access to public spaces that are unavailable to Stormfront users’ overt expressions of racism.[47]
Common natural language processing analysis blurs the distinction between white supremacist and mainstream speech
The regular processes of preparing text for analysis make recognition even harder. For example, many models do not recognize “Blacks” as a plural noun. Large language models (LLMs) are machine-learning models trained on massive quantities of text (such as millions of pages from English Wikipedia[48] or web-crawler data[49]). LLMs trained on general text from Wikipedia and the web expect “Blacks” to be a possessive proper noun, not a plural common noun, because the possessive proper form is more common in mainstream text. The information that would help a model distinguish “Blacks” from “Black’s” gets lost in common data-preparation steps in machine learning.
Before text is passed to machine-learning models, it is “preprocessed.” Preprocessing transforms the raw data into data suitable for whatever model is being trained or deployed. It includes steps such as converting all text to lowercase, removing common words that don’t carry much information, or modifying nearly identical words to appear the same. One of the primary goals of preprocessing is to reduce the overall size of the vocabulary the models need to use, but in reducing the size of vocabularies, we run the risk of removing rare but informative words or features. For instance, two common preprocessing steps, stemming and lemmatizing,[50] modify the words that appear in the text. Both stemming and lemmatizing are meant to reduce some variance in the words that we see and thus revise words to their base or root forms (for instance, the root form of “reading” is “read”). The problem is that when we stem or lemmatize a word like “Blacks,” it becomes its root form, “Black.” “Black” is most commonly used as an adjective, so a common analysis workflow changes both the part of speech and the number of the noun. Newer approaches to preparing text for analysis that use other methods of vocabulary reduction, such as WordPiece, run the same risk of collapsing plural nouns or losing information from very rare words[51], but they can be tuned to learn that “Blacks” is related to “Black” and not a wholly unique word. Because the differences between mainstream and extremist speech are so subtle, keeping markers such as plural nouns is essential to being able to tell the two types of speech apart.
Other techniques from machine learning (specifically, part-of-speech tagging and word embedding) also help to get information about the texts, such as the part of speech of a particular word or which words appear with it. For example, on Reddit, the words “Jew,”“evangelical,” “gay,” and “Stalin” often appeared together, while on Stormfront, the most common words to appear with “Jew” were the chunks Bernie [in] red, goy, and girls [are/were] raped. This example illustrates that content moderation tools that rely on words alone do not work. In another example, think about common words like “hoe” or “ape” that have both ordinary and derogatory meanings. Platforms need to incorporate information about words and their context into their mitigation approaches by including these specific linguistic markers.
Language models need more examples of white supremacists’ content to to catch their subtle linguistic differences
Computational tools trained on mainstream text don’t recognize the particulars of white supremacist speech. One approach platforms and researchers take to address these challenges is to use large language models (LLMs).[52] LLMs contribute to advances in many language tasks such as text summarization and chatbots, but they are also used in content moderation. LLMs are usually general models that perform well on general tasks. However, they are not always good candidates for addressing the specifics of detecting and mitigating white supremacy. LLMs exhibit biases related to race, gender, and religion[53], because they parrot stereotypes that appear in the data used to train them. In a critique of LLMs, researchers explained how “describing a woman’s account of her experience of sexism with the word tantrum both reflects a worldview where the sexist actions are normative and foregrounds a stereotype of women as childish and not in control of their emotions.”[54] The general language of the internet is biased against historically marginalized groups, and models trained using this language are as well.
LLMs are also not always explainable. Because they can incorporate so many dimensions and use multiple layers of analysis, LLMs can become black boxes, and it’s impossible to understand how they make decisions. Understanding how a model decides whether some content is acceptable is important for establishing trust and transparency in content-moderation decisions.
This bias and opacity means that general LLMs are not always effective tools for detecting white supremacy. LLMs can be tuned to address more specific goals. One way to overcome their challenges is to incorporate more data that contain the particulars of white supremacist speech. Recent research suggests that even small, curated datasets can improve LLM performance significantly.[55] Platforms must tune their LLMs or augment them to include more data from white supremacists to improve their ability to detect dangerous speech.
Conclusions
Overall, our findings indicate that white supremacist speech is identifiable and that the white supremacists in our sample talk about a consistent set of topics. Using existing text-comparison and topic-modeling approaches, we identified linguistic markers and subjects of conversation that can readily distinguish white supremacist language. We found that these markers included plural noun forms of racial and ethnic labels and “white” as an adjective for nouns with no explicit racial connotations, and that the topics of politics, culture, and explicit white supremacy separate white supremacist posts from general posts.
We recognize a number of limitations within our study. First, the samples of data we examined are small relative to all of Stormfront, Reddit, and Twitter. Examining broader sets of users or other platforms may reveal different linguistic patterns or suggest more diversity in their topics of conversation. Our findings are a first step in capturing the apparent differences between white supremacists and other users. Second, we used off-the-shelf computational techniques that are relatively blunt instruments. The simplicity of our approaches meant that we used only commercial and academic computing resources and a small team to conduct our study. Additional computational and human resources would afford more sophisticated analyses and may reveal additional distinguishing features and patterns. Future research can employ additional or alternative datasets and state-of-the-art computational approaches to build upon our findings.
We found that, even with more sophisticated computing capabilities and additional data, social media platforms miss a lot of white supremacist content. Their content-moderation processes especially struggle to distinguish non-profane yet hateful speech from profane but otherwise innocuous speech. Mainstream platform-moderation approaches neither capture the nuances of white supremacist speech nor leverage information provided by the unique features of white supremacist language. Using techniques that preserve the information available, such as plural noun forms and adjective phrases, leveraging more specific training datasets, and reducing their emphasis on profanity can improve platforms’ performance.
[38] Hatebase, Inc., Hatebase, 2019, https://hatebase.org/.
[39] Thomas Davidson et al., “Automated Hate Speech Detection and the Problem of Offensive Language,” in Eleventh International AAAI Conference on Web and Social Media, 2017, https://aaai.org/ocs/index.php/ICWSM/ICWSM17/paper/viewPaper/15665; Pete Burnap and Matthew L. Williams, “Cyber Hate Speech on Twitter: An Application of Machine Classification and Statistical Modeling for Policy and Decision Making,” Policy & Internet 7, no. 2 (2015): 223–42, https://onlinelibrary.wiley.com/doi/abs/10.1002/poi3.85; Irene Kwok and Yuzhou Wang, “Locate the Hate: Detecting Tweets against Blacks,” in Proceedings of the Twenty-Seventh AAAI Conference on Artificial Intelligence (Association for the Advancement of Artificial Intelligence, 2013), 1621–22, http://www.aaai.org/ocs/index.php/AAAI/AAAI13/paper/download/6419/6821; Shervin Malmasi and Marcos Zampieri, “Detecting Hate Speech in Social Media,” arXiv [cs.CL] (December 18, 2017), arXiv, http://arxiv.org/abs/1712.06427; Lei Gao, Alexis Kuppersmith, and Ruihong Huang, “Recognizing Explicit and Implicit Hate Speech Using a Weakly Supervised Two-Path Bootstrapping Approach,” in Proceedings of the Eighth International Joint Conference on Natural Language Processing (Volume 1: Long Papers) (Asian Federation of Natural Language Processing, 2017), 774–82, https://aclanthology.org/I17-1078/; Alexandra A. Siegel et al., “Trumping Hate on Twitter? Online Hate Speech in the 2016 U.S. Election Campaign and Its Aftermath,” Quarterly Journal of Political Science 16, no. 1 (2021): 71–104, https://doi.org/10.1561/100.00019045.
[40] Zeerak Waseem, “Are You a Racist or Am I Seeing Things? Annotator Influence on Hate Speech Detection on Twitter,” in Proceedings of the First Workshop on NLP and Computational Social Science (aclweb.org, 2016), 138–42, https://www.aclweb.org/anthology/W16-5618.
[41] Aymé Arango, Jorge Pérez, and Barbara Poblete, “Hate Speech Detection Is Not as Easy as You May Think: A Closer Look at Model Validation,” in Proceedings of the 42nd International ACM SIGIR Conference on Research and Development in Information Retrieval, SIGIR’19 (New York, NY, USA: Association for Computing Machinery, 2019), 45–54, https://doi.org/10.1145/3331184.3331262.
[42] Ona de Gibert et al., “Hate Speech Dataset from a White Supremacy Forum,” in Proceedings of the Second Workshop on Abusive Language Online (ALW2), 2018, 11–20, http://arxiv.org/abs/1809.04444.
[43] Rishav Hada et al., “Ruddit: Norms of Offensiveness for English Reddit Comments,” in Proceedings of the 59th Annual Meeting of the Association for Computational Linguistics and the 11th International Joint Conference on Natural Language Processing (Volume 1: Long Papers) (Online: Association for Computational Linguistics, 2021), 2700–2717, https://doi.org/10.18653/v1/2021.acl-long.210.
[44] Hatebase, Inc.
[45] See, for example, Leandro Silva et al., “Analyzing the Targets of Hate in Online Social Media,” in Proceedings of ICWSM, 2016, https://www.aaai.org/ocs/index.php/ICWSM/ICWSM16/paper/viewPaper/13147; Mai ElSherief et al., “Hate Lingo: A Target-Based Linguistic Analysis of Hate Speech in Social Media,” in Twelfth International AAAI Conference on Web and Social Media, 2018, http://arxiv.org/abs/1804.04257; Davidson et al., “Automated Hate Speech Detection and the Problem of Offensive Language”; Ping Liu et al., “Forecasting the Presence and Intensity of Hostility on Instagram Using Linguistic and Social Features,” Proceedings of the Twelfth International AAAI Conference on Web and Social Media (ICWSM 2018) 12, no. 1 (2018): 181–90, https://www.aaai.org/ocs/index.php/ICWSM/ICWSM18/paper/download/17875/17009.
[46] “Perspective API - How It Works,” accessed January 4, 2022, https://www.perspectiveapi.com/how-it-works/.
[47] Simi and Futrell, American Swastika: Inside the White Power Movement’s Hidden Spaces of Hate; Daniels, Cyber Racism: White Supremacy Online and the New Attack on Civil Rights.
[48] Devlin et al., “BERT: Pre-Training of Deep Bidirectional Transformers for Language Understanding.”
[49] Brown et al., “Language Models Are Few-Shot Learners.”
[50] “Stemming” reduces words to their stems or roots, e.g., from “acting” to “act;” “lemmatizing” reduces words to their meaningful bases or lemmas, e.g., from “actor’s” to “actor.”
[51] Coleman Haley, “This Is a BERT. Now There Are Several of Them. Can They Generalize to Novel Words?,” in Proceedings of the Third BlackboxNLP Workshop on Analyzing and Interpreting Neural Networks for NLP (Online: Association for Computational Linguistics, 2020), 333–41, https://doi.org/10.18653/v1/2020.blackboxnlp-1.31.
[52] Karen Hao, “The Race to Understand the Exhilarating, Dangerous World of Language AI,” MIT Technology Review, May 20, 2021, https://www.technologyreview.com/2021/05/20/1025135/ai-large-language-models-bigscience-project/.
[53] Emily M. Bender et al., “On the Dangers of Stochastic Parrots: Can Language Models Be Too Big? 🦜,” in Proceedings of the 2021 ACM Conference on Fairness, Accountability, and Transparency, FAccT ’21 (New York, NY, USA: Association for Computing Machinery, 2021), 610–23, https://doi.org/10.1145/3442188.3445922; Alex Tamkin et al., “Understanding the Capabilities, Limitations, and Societal Impact of Large Language Models,” arXiv [cs.CL] (February 4, 2021), arXiv, http://arxiv.org/abs/2102.02503; Yi Chern Tan and L. Elisa Celis, “Assessing Social and Intersectional Biases in Contextualized Word Representations,” Advances in Neural Information Processing Systems, November 4, 2019, 13230–41, http://arxiv.org/abs/1911.01485.
[54] Bender et al., “On the Dangers of Stochastic Parrots: Can Language Models Be Too Big? 🦜.”
[55] Irene Solaiman and Christy Dennison, “Process for Adapting Language Models to Society (PALMS) with Values-Targeted Datasets,” arXiv [cs.CL] (June 18, 2021), arXiv, https://cdn.openai.com/palms.pdf.
Recommendations
Platform Recommendations
Enforce existing rules equitably and at scale
Most mainstream social media platforms already have rules against hate speech, hateful images, and hateful conduct.[56] Their policies are not consistently or transparently enforced, however. For instance, BIPOC and LGBTQ+ users as well as civil rights groups have long been vocal about how Facebook’s automated systems unfairly remove content criticizing discrimination and others’ hateful actions but allow white supremacist content to thrive.[57] ADL has similarly found that major platforms fail to catch and remove large swaths of antisemitic content, such as Holocaust denial, as ADL’s recent report cards show. Facebook, meanwhile, recently came under fire for its XCheck (pronounced “crosscheck”) system, which allowed millions of users with large followings, from celebrities and influencers to political leaders, to post content that regular users cannot by exempting them from typical AI detection and content moderation.[58]
Setting aside whether the platforms’ policies include the correct set of rights and responsibilities for users, unfair enforcement of those rules undermines their effectiveness. Procedural justice, which includes both fair decisions and fair treatment,[59] is a useful framework for thinking about how to encourage cooperation with platform policies.[60] According to this framework, people defer to the rules when they perceive them as fairly determined and fairly applied.[61] Researchers suggest that lessons from criminal justice reform can improve design processes for social media platforms to help increase compliance and reduce recidivism.[62] Recently, an experiment about procedural justice on social media platforms confirmed that sanctioned users who perceived the process as evenhanded were less likely to violate policies in the future.[63] These findings and successes in other justice systems suggest that impartial enforcement can help social media platforms to ensure that users follow their existing guidelines.
Train and tune detection models with data from extremist sites
Seeding supervised algorithms with data from extremist sites is one avenue for detecting white supremacist language on mainstream sites, which researchers can do by scraping publicly accessible sites like Stormfront. As noted earlier, general language models are not designed to be able to detect the subtle differences or rare occurrences of white supremacist speech. Luckily, tuning models to recognize specific types of content is possible.[64] Resources such as Stormfront, NS88.com, white nationalist publications, and white power music contain many examples of white supremacist speech that can be readily accessed and used to tune more specific language models.
Platforms need new ways to incorporate information about words and their context into their mitigation approaches. As described earlier, word embeddings are one approach to providing additional input to models that capture the context of a specific word. As this report demonstrates, content moderation that relies on words alone does not work.
In addition to word embeddings, increases in computational power make it possible to represent larger regions of text in embeddings as well.[65] These advances mean that even more context than just individual or nearby words can be included in training data. For example, in an earlier section, we included a tweet of a conspiracy theory about Jews and the media. Instead of representing only the individual words in a tweet, it’s now possible to represent the whole tweet in a single embedding so that a longer sequence of words can be understood as a phrase. In that example, the phrase “Chief Rabbi Complains that Jews No Longer Have a Media Monopoly Because of the Free internet” becomes the object to be represented rather than each word or pair being a separate object. These larger, more complex representations capture more nuance and context, potentially improving detection.
Include specific linguistic markers in detection algorithms
In addition to training and tuning models with data from extremist sites, platforms should explicitly include readily identifiable features of white supremacist speech such as plural group nouns in their detection algorithms. Explicit white supremacy is easy to spot in both specific language and broad themes. Such speech often centers on discussions of race and “whites,” uses distinctive adjectives and plural noun forms, and invokes conspiracy theories. Platforms should invest more into creating, updating, and maintaining tools to identify such language.
Advances in machine learning have changed the requirements for preprocessing text. Many preprocessing steps are designed to reduce the computational overhead of training or applying a model—for instance, stemmed words require fewer dimensions than unstemmed words. That means “Blacks” and “Black’s” can each be included in the training data for a model; it’s no longer necessary to collapse them both to “Black” and lose the information contained in the plural and possessive forms of the word. Because of these advances, adding these unique features of white supremacist speech to the training data for models has the potential to further improve their ability to detect subtly different and dangerous speech.
Preprocessing steps are often designed to reduce the dimensionality or complexity of data before modeling. Feature selection, the process of deciding which measurable aspects of data to use in the computational model, further reduces dimensions.[66] Social media data has so many dimensions—the individual words, the words and phrases around them, the author and their properties, etc.—that could serve as useful features. Feature selection reduces these dimensions to simplify and speed up models. Because there are common, recognizable features of white supremacist speech, platforms can be smart about choosing those features to include in their models. The presence of “white” in adjective form modifying nouns like “genocide,” for instance, is a measurable feature. Plural noun forms of racial and ethnic markers (Blacks, Jews) are other features we can select. Combining (1) better training data, (2) improved data-representation, and (3) smart feature-selection is a way to improve the machine-learning components of detection mechanisms.
De-emphasize profanity in toxicity detection
Platforms should give less weight to profanity in white supremacy detection approaches.[67] Feature selection doesn’t require that features be positively weighted; we can also indicate features, like profane words, that can be negatively associated with the data we want to identify. That means we can positively weight “white genocide” and negatively weight “shitpost” to increase the likelihood that white supremacist speech gets flagged for review or removal while common internet profanity is ignored. Our findings show that white supremacists use “civil” or polite language. Platforms can adjust their attention accordingly toward polite but hateful content.
Train moderators and algorithms to recognize the dangers of white power conversations
Platforms should train their moderators, both paid and unpaid, to recognize conversations that praise or recommend white supremacist ideology. Facebook uses its own designations for hate organizations, but it should also adopt definitions and leverage knowledge from outside organizations such as ADL.[68] Existing knowledge from civil rights groups could teach moderators how to recognize symbols appropriated by hate groups and distinguish legitimate debate from insincere trolling. Experts are better equipped to detect subtle differences, memes, and aggression,[69] and platforms should also help their moderators gain the necessary expertise, for example, through training programs with civil society organizations. Platforms must also increase their own internal expertise on specific forms of hate; they cannot rely on external organizations alone.
Trained moderators should also be able to identify white supremacist activity outside of the text of social media posts. For example, we noted that white supremacist language occurs in user names (“Gaston Chambers”) and profiles. Others have highlighted the ways that white supremacists adapt images[70] and video games[71] to spread hate. Platforms’ efforts to address white supremacy must attend to all forms of user-generated content, not just the written content of their posts. As one internal audit of Facebook’s civil rights record makes clear,[72] addressing explicit white supremacy, on one hand, and praise or support for white supremacist content on mainstream platforms, on the other, requires different mechanisms. Improving moderator training and attending to all forms of user-generated content are promising avenues toward improving detection and mitigation methods.
Government and Policy Recommendations
ADL’s REPAIR Plan is a comprehensive framework to decrease hate online and push extremism back to the fringes of the digital world. In line with REPAIR, we encourage governments to:
Change platform incentive systems by updating regulations and reforming existing laws
Congress must effectively reform, not eliminate, Section 230 of the Communications Decency Act to hold social media platforms accountable for their role in fomenting violence, extremist disinformation, and other forms of hate leading to harm—especially because of Big Tech’s algorithmic amplification of dangerous content. Reform, however, must prioritize civil rights and civil liberties concerns rather than overbroadly suppressing free speech or unintentionally cementing the monopolistic power of Big Tech by making it too costly for all but the largest platforms to ward off frivolous lawsuits and trolls.
Prioritize systematized, comprehensive, and easily accessible transparency
Platforms claim to have strong policies against hate, gender-based violence, and extremism, when in fact, most are unclear, hard to find, or have perplexing exceptions. Enforcement is inequitable and inconsistent, and transparency reports are incomplete, irregular, and opaque. Policymakers must pass laws and undertake approaches that require regular reporting, increased transparency, and independent audits regarding content moderation, algorithms, and engagement features while looking for other incentive-based or regulatory action. Platform transparency reporting must evaluate success and provide evidence that independent researchers can use. Such independent researchers must be granted access to data, including archives of moderated content, and Congress must have an oversight role.
Provide more resources for investigating cyberstalking, doxxing, and swatting and increase support for targets of cyberhate
Additionally, Congress should update gaps and loopholes in cyberharassment laws and the reporting of bias-based digital abuse in order to better protect victims and targets, including enacting legislation related to doxxing, swatting, and non-consensual distribution of intimate imagery. According to ADL’s ethnographic study of online hate and harassment, “some of the most widely reported incidents of campaign harassment (the ability of harassers to use online networks to organize campaigns of hate) and networked harassment (the weaponization of a target’s online network) have been waged against women and the LGBTQ+ community.” Victims and targets of cyberhate need more resources and support. Congress and the administration should work together to create a resource center to support targets of identity-based online harassment. This center could provide tools to victims and targets seeking to communicate with social media platforms, report unlawful behavior to law enforcement, and receive extra care. Additionally, creating a hotline for victims and targets of cyber-hate and harassment and requiring platforms to regularly report on the quantity and types of hate and harassment that were reported and actioned can help us to tackle this issue.
Support research and innovation
Governments must focus on research and innovation to slow the spread of online hate, including but not limited to: (1) measurement of online hate; (2) hate and extremism in online games; (3) methods of off-ramping vulnerable individuals who have been radicalized; (4) the connection between online hate speech and hate crimes; (5) new methods of disinformation; (6) the role of internet infrastructure providers and online funding sources in supporting and facilitating the spread of hate and extremism; (7) the role of monopolistic power in spreading online hate; (8) audio and video content moderation. Researching areas like these is crucial to developing innovative yet sustainable solutions to decrease online hate.
[56] See e.g.,“Hate Speech,” accessed January 4, 2022; “Hateful Conduct Policy,” Twitter, n.d.; “Content Policy - Reddit,” accessed January 4, 2022.
[57] Laura W. Murphy and Relman Colfax, “Facebook’s Civil Rights Audit – Final Report” (pdf), July 8, 2020.
[58] Elizabeth Dwoskin, Craig Timberg, and Tony Romm, “Zuckerberg Once Wanted to Sanction Trump. Then Facebook Wrote Rules That Accommodated Him,” The Washington Post, June 28, 2020; Jeff Horwitz, “Facebook Says Its Rules Apply to All. Company Documents Reveal a Secret Elite That’s Exempt,” WSJ Online, September 13, 2021.
[59] Steven L. Blader and Tom R. Tyler, “A Four-Component Model of Procedural Justice: Defining the Meaning of a ‘Fair’ Process,” Personality & Social Psychology Bulletin 29, no. 6 (June 1, 2003): 747–58, https://doi.org/10.1177/0146167203029006007.
[60] David Jurgens, Eshwar Chandrasekharan, and Libby Hemphill, “A Just and Comprehensive Strategy for Using NLP to Address Online Abuse,” in Proceedings of the 57th Annual Meeting of the Association for Computational Linguistics (ACL), (57th Annual Meeting of the Association for Computational Linguistics (ACL), 2019) (57th Annual Meeting of the Association for Computational Linguistics (ACL), 2019), https://www.aclweb.org/anthology/P19-1357.pdf.
[61] Tom R. Tyler, Phillip Atiba Goff, and Robert J. MacCoun, “The Impact of Psychological Science on Policing in the United States: Procedural Justice, Legitimacy, and Effective Law Enforcement,” Psychological Science in the Public Interest: A Journal of the American Psychological Society 16, no. 3 (December 2015): 75–109, https://doi.org/10.1177/1529100615617791.
[62] Jurgens, Chandrasekharan, and Hemphill, “A Just and Comprehensive Strategy for Using NLP to Address Online Abuse”; Siapera and Viejo-Otero, “Governing Hate: Facebook and Digital Racism”; Sarita Schoenebeck, Oliver L. Haimson, and Lisa Nakamura, “Drawing from Justice Theories to Support Targets of Online Harassment,” New Media & Society, March 25, 2020, 1461444820913122, https://doi.org/10.1177/1461444820913122.
[63] Tom Tyler et al., “Social Media Governance: Can Social Media Companies Motivate Voluntary Rule Following Behavior among Their Users?,” Journal of Experimental Criminology 17, no. 1 (March 1, 2021): 109–27, https://doi.org/10.1007/s11292-019-09392-z.
[64] See, e.g., Jeremy Howard and Sebastian Ruder, “Universal Language Model Fine-Tuning for Text Classification,” arXiv [cs.CL] (January 18, 2018), arXiv, http://arxiv.org/abs/1801.06146; Rie Johnson and Tong Zhang, “Supervised and Semi-Supervised Text Categorization Using LSTM for Region Embeddings,” in Proceedings of The 33rd International Conference on Machine Learning, ed. Maria Florina Balcan and Kilian Q. Weinberger, vol. 48, Proceedings of Machine Learning Research (New York, New York, USA: PMLR, 2016), 526–34, https://proceedings.mlr.press/v48/johnson16.html; Bjarke Felbo et al., “Using Millions of Emoji Occurrences to Learn Any-Domain Representations for Detecting Sentiment, Emotion and Sarcasm,” arXiv [stat.ML] (August 1, 2017), arXiv, http://arxiv.org/abs/1708.00524.
[65] Johnson and Zhang, “Supervised and Semi-Supervised Text Categorization Using LSTM for Region Embeddings.”
[66] Anirban Dasgupta et al., “Feature Selection Methods for Text Classification,” in Proceedings of the 13th ACM SIGKDD International Conference on Knowledge Discovery and Data Mining, KDD ’07 (New York, NY, USA: Association for Computing Machinery, 2007), 230–39, https://doi.org/10.1145/1281192.1281220; Avrim L. Blum and Pat Langley, “Selection of Relevant Features and Examples in Machine Learning,” Artificial Intelligence 97, no. 1 (December 1, 1997): 245–71, https://doi.org/10.1016/S0004-3702(97)00063-5.
[67] Libby Hemphill, “More Specificity, More Attention to Social Context: Reframing How We Address ‘Bad Actors,’” arXiv [cs.SI] (February 23, 2018), arXiv, http://arxiv.org/abs/1802.08612.
[68] Tech Transparency Project, “White Supremacist Groups Are Thriving on Facebook” (pdf), May 21, 2020.
[69] Hala Al Kuwatly, Maximilian Wich, and Georg Groh, “Identifying and Measuring Annotator Bias Based on Annotators’ Demographic Characteristics,” in Proceedings of the Fourth Workshop on Online Abuse and Harms (aclweb.org, 2020), 184–90, https://www.aclweb.org/anthology/2020.alw-1.21/; Waseem, “Are You a Racist or Am I Seeing Things? Annotator Influence on Hate Speech Detection on Twitter.”
[70] Daniels, Cyber Racism: White Supremacy Online and the New Attack on Civil Rights; Simi and Futrell, American Swastika: Inside the White Power Movement’s Hidden Spaces of Hate.
[71] Anti-Defamation League, “Disruption and Harms in Online Gaming Framework."
[72] Murphy and Colfax, “Facebook’s Civil Rights Audit – Final Report.”
References
Al Kuwatly, Hala, Maximilian Wich, and Georg Groh. “Identifying and Measuring Annotator Bias Based on Annotators’ Demographic Characteristics.” In Proceedings of the Fourth Workshop on Online Abuse and Harms, 184–90. aclweb.org, 2020. https://www.aclweb.org/anthology/2020.alw-1.21/.
Anti-Defamation League. “Alt Right: A Primer on the New White Supremacy.” Accessed October 20, 2021. https://www.adl.org/resources/backgrounders/alt-right-a-primer-on-the-new-white-supremacy.
Anti-Defamation League. “The Extremist Medicine Cabinet: A Guide to Online ‘Pills,’” November 2019. https://www.adl.org/blog/the-extremist-medicine-cabinet-a-guide-to-online-pills.
Arango, Aymé, Jorge Pérez, and Barbara Poblete. “Hate Speech Detection Is Not as Easy as You May Think: A Closer Look at Model Validation.” In Proceedings of the 42nd International ACM SIGIR Conference on Research and Development in Information Retrieval, 45–54. SIGIR’19. New York, NY, USA: Association for Computing Machinery, 2019. https://doi.org/10.1145/3331184.3331262.
Bender, Emily M., Timnit Gebru, Angelina McMillan-Major, and Shmargaret Shmitchell. “On the Dangers of Stochastic Parrots: Can Language Models Be Too Big? 🦜.” In Proceedings of the 2021 ACM Conference on Fairness, Accountability, and Transparency, 610–23. FAccT ’21. New York, NY, USA: Association for Computing Machinery, 2021. https://doi.org/10.1145/3442188.3445922.
Berger, J. M. “The Alt-Right Twitter Census: Defining and Describing the Audience for Alt-Right Content on Twitter.” VOX-Pol Network of Excellence, 2018. https://www.voxpol.eu/download/vox-pol_publication/AltRightTwitterCensus.pdf.
Blader, Steven L., and Tom R. Tyler. “A Four-Component Model of Procedural Justice: Defining the Meaning of a ‘Fair’ Process.” Personality & Social Psychology Bulletin 29, no. 6 (June 1, 2003): 747–58. https://doi.org/10.1177/0146167203029006007.
Blum, Avrim L., and Pat Langley. “Selection of Relevant Features and Examples in Machine Learning.” Artificial Intelligence 97, no. 1 (December 1, 1997): 245–71. https://doi.org/10.1016/S0004-3702(97)00063-5.
Bourdieu, Pierre. Language and Symbolic Power. Cambridge, MA, USA: Harvard University Press, 1991.
Breland, Ali. “Reddit Finally Banned The_Donald. That Won’t Stop the Hate It Unleashed.” Mother Jones. Accessed January 4, 2022. https://www.motherjones.com/politics/2020/06/reddit_the_donald_ban/.
Brock, André. “Beyond the Pale: The Blackbird Web Browser’s Critical Reception.” New Media & Society 13, no. 7 (November 1, 2011): 1085–1103. https://doi.org/10.1177/1461444810397031.
Bucholtz, Mary. “The Public Life of White Affects.” Journal of Sociolinguistics 23, no. 5 (November 2019): 485–504. https://doi.org/10.1111/josl.12392.
Burnap, Pete, and Matthew L. Williams. “Cyber Hate Speech on Twitter: An Application of Machine Classification and Statistical Modeling for Policy and Decision Making.” Policy & Internet 7, no. 2 (2015): 223–42. https://onlinelibrary.wiley.com/doi/abs/10.1002/poi3.85.
Anti-Defamation League. “The Online Hate Index: Innovation Brief.” January 2018. https://www.adl.org/resources/reports/the-online-hate-index.
“Content Policy - Reddit.” Accessed January 4, 2022. https://www.redditinc.com/policies/content-policy.
Cope, Sophia, Jillian C. York, and Jeremy Gillula. “Industry Efforts to Censor Pro-Terrorism Online Content Pose Risks to Free Speech.” Electronic Frontier Foundation, July 12, 2017. https://perma.cc/NUM5-Q9HY.
Daniels, Jessie. Cyber Racism: White Supremacy Online and the New Attack on Civil Rights. Lanham, MD: Rowman & Littlefield Publishers, 2009.
———. “The Algorithmic Rise of the ‘Alt-Right.’” Contexts 17, no. 1 (February 1, 2018): 60–65. https://doi.org/10.1177/1536504218766547.
Dasgupta, Anirban, Petros Drineas, Boulos Harb, Vanja Josifovski, and Michael W. Mahoney. “Feature Selection Methods for Text Classification.” In Proceedings of the 13th ACM SIGKDD International Conference on Knowledge Discovery and Data Mining, 230–39. KDD ’07. New York, NY, USA: Association for Computing Machinery, 2007. https://doi.org/10.1145/1281192.1281220.
Davidson, Thomas, Dana Warmsley, Michael Macy, and Ingmar Weber. “Automated Hate Speech Detection and the Problem of Offensive Language.” In Eleventh International AAAI Conference on Web and Social Media, 2017. https://aaai.org/ocs/index.php/ICWSM/ICWSM17/paper/viewPaper/15665.
DeCook, Julia R. “Memes and Symbolic Violence: #proudboys and the Use of Memes for Propaganda and the Construction of Collective Identity.” Learning, Media and Technology 43, no. 4 (October 2, 2018): 485–504. https://doi.org/10.1080/17439884.2018.1544149.
Duggan, Maeve. “Online Harassment 2017.” Pew Research Center, 2017.
Dwoskin, Elizabeth, Craig Timberg, and Tony Romm. “Zuckerberg Once Wanted to Sanction Trump. Then Facebook Wrote Rules That Accommodated Him.” The Washington Post. June 28, 2020. https://www.washingtonpost.com/technology/2020/06/28/facebook-zuckerberg-trump-hate/.
ElSherief, Mai, Vivek Kulkarni, Dana Nguyen, William Yang Wang, and Elizabeth Belding. “Hate Lingo: A Target-Based Linguistic Analysis of Hate Speech in Social Media.” In Twelfth International AAAI Conference on Web and Social Media, 2018. http://arxiv.org/abs/1804.04257.
Felbo, Bjarke, Alan Mislove, Anders Søgaard, Iyad Rahwan, and Sune Lehmann. “Using Millions of Emoji Occurrences to Learn Any-Domain Representations for Detecting Sentiment, Emotion and Sarcasm.” arXiv [stat.ML], August 1, 2017. arXiv. http://arxiv.org/abs/1708.00524.
Fernandez, Miriam, Moizzah Asif, and Harith Alani. “Understanding the Roots of Radicalisation on Twitter.” In Proceedings of the 10th ACM Conference on Web Science, 1–10. WebSci ’18. New York, NY, USA: Association for Computing Machinery, 2018. https://doi.org/10.1145/3201064.3201082.
Fluri, Jennifer, and Lorraine Dowler. “House Bound: Women’s Agency in White Separatist Movements.” In Spaces of Hate: Geographies of Discrimination and Intolerance in the U. S. A., edited by Colin Flint, 69–85. London, UK: Taylor & Francis Group, 2003.
Gallaher, Carolyn. “Mainstreaming White Supremacy: A Twitter Analysis of the American ‘Alt-Right.’” Gender, Place and Culture: A Journal of Feminist Geography 28, no. 2 (February 1, 2021): 224–52. https://doi.org/10.1080/0966369X.2019.1710472.
Gao, Lei, Alexis Kuppersmith, and Ruihong Huang. “Recognizing Explicit and Implicit Hate Speech Using a Weakly Supervised Two-Path Bootstrapping Approach.” In Proceedings of the Eighth International Joint Conference on Natural Language Processing (Volume 1: Long Papers), 774–82. Asian Federation of Natural Language Processing, 2017. https://aclanthology.org/I17-1078/.
Gibert, Ona de, Naiara Perez, Aitor García-Pablos, and Montse Cuadros. “Hate Speech Dataset from a White Supremacy Forum.” In Proceedings of the Second Workshop on Abusive Language Online (ALW2), 11–20, 2018. http://arxiv.org/abs/1809.04444.
Gillespie, Tarleton. “The Politics of ‘platforms.’” New Media & Society 12, no. 3 (2010): 347–64. http://nms.sagepub.com/content/12/3/347.short.
“Goblin - WoW.” Accessed January 4, 2022. https://worldofwarcraft.com/en-us/game/races/goblin.
Hada, Rishav, Sohi Sudhir, Pushkar Mishra, Helen Yannakoudakis, Saif M. Mohammad, and Ekaterina Shutova. “Ruddit: Norms of Offensiveness for English Reddit Comments.” In Proceedings of the 59th Annual Meeting of the Association for Computational Linguistics and the 11th International Joint Conference on Natural Language Processing (Volume 1: Long Papers), 2700–2717. Online: Association for Computational Linguistics, 2021. https://doi.org/10.18653/v1/2021.acl-long.210.
Haley, Coleman. “This Is a BERT. Now There Are Several of Them. Can They Generalize to Novel Words?” In Proceedings of the Third Blackbox NLP Workshop on Analyzing and Interpreting Neural Networks for NLP, 333–41. Online: Association for Computational Linguistics, 2020. https://doi.org/10.18653/v1/2020.blackboxnlp-1.31.
Hao, Karen. “The Race to Understand the Exhilarating, Dangerous World of Language AI.” MIT Technology Review, May 20, 2021. https://www.technologyreview.com/2021/05/20/1025135/ai-large-language-models-bigscience-project/.
Hatebase, Inc. Hatebase, 2019. https://hatebase.org/.
Twitter. “Hateful Conduct Policy,” n.d. https://help.twitter.com/en/rules-and-policies/hateful-conduct-policy.
“Hate Speech.” Accessed January 4, 2022. https://transparency.fb.com/policies/community-standards/hate-speech/.
Hemphill, Libby. “More Specificity, More Attention to Social Context: Reframing How We Address ‘Bad Actors.’” arXiv [cs.SI], February 23, 2018. arXiv. http://arxiv.org/abs/1802.08612.
Hemphill, Libby, Annelise Russell, and Angela M. Schöpke-Gonzalez. “What Drives U.S. Congressional Members’ Policy Attention on Twitter?” Policy & Internet, 2020. https://doi.org/10.1002/poi3.245.
Hemphill, Libby, and Angela M. Schöpke-Gonzalez. “Two Computational Models for Analyzing Political Attention in Social Media.” Proceedings of the AAAI Conference on Web and Social Media 14, no. 1 (2020): 260–71. https://www.aaai.org/ojs/index.php/ICWSM/article/view/7297.
Horwitz, Jeff. “Facebook Knew Calls for Violence Plagued ‘Groups’, Now Plans Overhaul.” WSJ Online, January 31, 2021. https://www.wsj.com/articles/facebook-knew-calls-for-violence-plagued-groups-now-plans-overhaul-11612131374.
———. “Facebook Says Its Rules Apply to All. Company Documents Reveal a Secret Elite That’s Exempt.” WSJ Online, September 13, 2021. https://www.wsj.com/articles/facebook-files-xcheck-zuckerberg-elite-rules-11631541353?mod=article_inline.
Howard, Jeremy, and Sebastian Ruder. “Universal Language Model Fine-Tuning for Text Classification.” arXiv [cs.CL], January 18, 2018. arXiv. http://arxiv.org/abs/1801.06146.
Johnson, Rie, and Tong Zhang. “Supervised and Semi-Supervised Text Categorization Using LSTM for Region Embeddings.” In Proceedings of The 33rd International Conference on Machine Learning, edited by Maria Florina Balcan and Kilian Q. Weinberger, 48:526–34. Proceedings of Machine Learning Research. New York, New York, USA: PMLR, 2016. https://proceedings.mlr.press/v48/johnson16.html.
Jurgens, David, Eshwar Chandrasekharan, and Libby Hemphill. “A Just and Comprehensive Strategy for Using NLP to Address Online Abuse.” In Proceedings of the 57th Annual Meeting of the Association for Computational Linguistics (ACL), 2019. https://www.aclweb.org/anthology/P19-1357.pdf.
Kwok, Irene, and Yuzhou Wang. “Locate the Hate: Detecting Tweets against Blacks.” In Proceedings of the Twenty-Seventh AAAI Conference on Artificial Intelligence, 1621–22. Association for the Advancement of Artificial Intelligence, 2013. http://www.aaai.org/ocs/index.php/AAAI/AAAI13/paper/download/6419/6821.
Liu, Ping, Joshua Guberman, Libby Hemphill, and Aron Culotta. “Forecasting the Presence and Intensity of Hostility on Instagram Using Linguistic and Social Features.” Proceedings of the Twelfth International AAAI Conference on Web and Social Media (ICWSM 2018) 12, no. 1 (2018): 181–90. https://www.aaai.org/ocs/index.php/ICWSM/ICWSM18/paper/download/17875/17009.
Magu, Rijul, Kshitij Joshi, and Jiebo Luo. “Detecting the Hate Code on Social Media.” In Eleventh International AAAI Conference on Web and Social Media, 2017. https://www.aaai.org/ocs/index.php/ICWSM/ICWSM17/paper/viewPaper/15604.
Magu, Rijul, and Jiebo Luo. “Determining Code Words in Euphemistic Hate Speech Using Word Embedding Networks.” Proceedings of the 2nd Workshop on Abusive Language Online, 2018. https://www.aclweb.org/anthology/W18-5112.pdf.
Malmasi, Shervin, and Marcos Zampieri. “Detecting Hate Speech in Social Media.” arXiv [cs.CL], December 18, 2017. arXiv. http://arxiv.org/abs/1712.06427.
Marantz, Andrew. “Why Facebook Can’t Fix Itself.” The New Yorker. October 9, 2020. https://www.newyorker.com/magazine/2020/10/19/why-facebook-cant-fix-itself.
Martin, James H., and Dan Jurafsky. Speech and Language Processing: An Introduction to Natural Language Processing, Computational Linguistics, and Speech Recognition. Upper Saddle River, N.J.: Prentice Hall, 2000.
Marwick, Alice, and Rebecca Lewis. “Media Manipulation and Disinformation Online.” Data & Society, May 15, 2017. https://datasociety.net/wp-content/uploads/2017/05/DataAndSociety_MediaManipulationAndDisinformationOnline-1.pdf.
Matamoros-Fernández, Ariadna. “Platformed Racism: The Mediation and Circulation of an Australian Race-Based Controversy on Twitter, Facebook and YouTube.” Information, Communication and Society 20, no. 6 (June 3, 2017): 930–46. https://doi.org/10.1080/1369118X.2017.1293130.
Murphy, Laura W., and Relman Colfax. “Facebook’s Civil Rights Audit – Final Report,” July 8, 2020. https://www.relmanlaw.com/media/cases/988_Civil-Rights-Audit-Final-Report.pdf.
Nakamura, Lisa. Cybertypes: Race, Ethnicity, and Identity on the Internet. Florence, UK: Routledge, 2013.
Paul, Kari. “‘It Let White Supremacists Organize’: The Toxic Legacy of Facebook’s Groups.” The Guardian. February 4, 2021. http://www.theguardian.com/technology/2021/feb/04/facebook-groups-misinformation.
“Perspective API - How It Works.” Accessed January 4, 2022. https://www.perspectiveapi.com/how-it-works/.
Řehůřek, Radim, and Petr Sojka. “Software Framework for Topic Modelling with Large Corpora.” In Proceedings of the LREC 2010 Workshop on New Challenges for NLP Frameworks, 45–50. Valletta, Malta: ELRA, 2010.
Schoenebeck, Sarita, Oliver L. Haimson, and Lisa Nakamura. “Drawing from Justice Theories to Support Targets of Online Harassment.” New Media & Society, March 25, 2020, 1461444820913122. https://doi.org/10.1177/1461444820913122.
Siapera, Eugenia, and Paloma Viejo-Otero. “Governing Hate: Facebook and Digital Racism.” Television & New Media 22, no. 2 (February 1, 2021): 112–30. https://doi.org/10.1177/1527476420982232.
Siegel, Alexandra A., Evgenii Nikitin, Pablo Barberá, Joanna Sterling, Bethany Pullen, Richard Bonneau, Jonathan Nagler, and Joshua A. Tucker. “Trumping Hate on Twitter? Online Hate Speech in the 2016 U.S. Election Campaign and Its Aftermath.” Quarterly Journal of Political Science 16, no. 1 (2021): 71–104. https://doi.org/10.1561/100.00019045.
Silva, Leandro, Mainack Mondal, Denzil Correa, Fabrício Benevenuto, and Ingmar Weber. “Analyzing the Targets of Hate in Online Social Media.” In Proceedings of ICWSM, 2016. https://www.aaai.org/ocs/index.php/ICWSM/ICWSM16/paper/viewPaper/13147.
Simi, Pete, and Robert Futrell. American Swastika: Inside the White Power Movement’s Hidden Spaces of Hate. Lanham, MD: Rowman & Littlefield Publishers, 2015. http://ebookcentral.proquest.com/lib/umichigan/detail.action?docID=2081815.
Solaiman, Irene, and Christy Dennison. “Process for Adapting Language Models to Society (PALMS) with Values-Targeted Datasets.” arXiv [cs.CL], June 18, 2021. arXiv. https://cdn.openai.com/palms.pdf.
Speakman, Burton, and Marcus Funk. “News, Nationalism, and Hegemony: The Formation of Consistent Issue Framing Throughout the U.S. Political Right.” Mass Communication and Society 23, no. 5 (September 2, 2020): 656–81. https://doi.org/10.1080/15205436.2020.1764973.
Stern, Alexandra Minna. Proud Boys and the White Ethnostate: How the Alt-Right Is Warping the American Imagination. Beacon Press, 2019.
Stevens, Francesca, Jason R. C. Nurse, and Budi Arief. “Cyber Stalking, Cyber Harassment, and Adult Mental Health: A Systematic Review.” Cyberpsychology, Behavior and Social Networking 24, no. 6 (June 2021): 367–76. https://doi.org/10.1089/cyber.2020.0253.
“Stop Hate for Profit.” Accessed January 4, 2022. https://www.stophateforprofit.org/.
Tamkin, Alex, Miles Brundage, Jack Clark, and Deep Ganguli. “Understanding the Capabilities, Limitations, and Societal Impact of Large Language Models.” arXiv [cs.CL], February 4, 2021. arXiv. http://arxiv.org/abs/2102.02503.
Tan, Yi Chern, and L. Elisa Celis. “Assessing Social and Intersectional Biases in Contextualized Word Representations.” Advances in Neural Information Processing Systems, November 4, 2019, 13230–41. http://arxiv.org/abs/1911.01485.
Tech Transparency Project. “White Supremacist Groups Are Thriving on Facebook,” May 21, 2020. https://www.techtransparencyproject.org/sites/default/files/Facebook-White-Supremacy-Report.pdf.
Tyler, Tom, Matt Katsaros, Tracey Meares, and Sudhir Venkatesh. “Social Media Governance: Can Social Media Companies Motivate Voluntary Rule Following Behavior among Their Users?” Journal of Experimental Criminology 17, no. 1 (March 1, 2021): 109–27. https://doi.org/10.1007/s11292-019-09392-z.
Tyler, Tom R., Phillip Atiba Goff, and Robert J. MacCoun. “The Impact of Psychological Science on Policing in the United States: Procedural Justice, Legitimacy, and Effective Law Enforcement.” Psychological Science in the Public Interest: A Journal of the American Psychological Society 16, no. 3 (December 2015): 75–109. https://doi.org/10.1177/1529100615617791.
Waseem, Zeerak. “Are You a Racist or Am I Seeing Things? Annotator Influence on Hate Speech Detection on Twitter.” In Proceedings of the First Workshop on NLP and Computational Social Science, 138–42. aclweb.org, 2016. https://www.aclweb.org/anthology/W16-5618.
“White Supremacy.” Accessed January 4, 2022. https://www.adl.org/resources/glossary-terms/white-supremacy.
Wilson, Richard Ashby, and Molly K. Land. “Hate Speech on Social Media: Content Moderation in Context.” Connecticut Law Review 52, no. 3 (2021): 1029–76. https://papers.ssrn.com/abstract=3690616.
Appendix: Methods in Detail
In this report, we compared content from three platforms—Stormfront, Twitter, and Reddit—to identify unique characteristics of white supremacist content and to measure its changes over time. We used web scraping to collect Stormfront data and the application programming interfaces (APIs) for both Twitter and Reddit. “Scraping” means that we used a computer program to mimic a human reading Stormfront and then downloaded the content we found. APIs are ways that platforms provide computers with programmatic access to their data. We sent queries for specific users and phrases to Twitter’s API, and it returned all tweets that matched. For Reddit, we randomly sampled 15,000 public comments/month for the time periods used in our analysis.
We used the Alt-Right Twitter Census[73] to identify accounts on Twitter to collect. In the census, researchers identified 27,895 accounts as “alt-right” accounts according to their Twitter profiles and the profiles of accounts they followed; we were only able to retrieve data from 2,237 accounts, for reasons that Twitter does not make clear. The term “alt-right” was adopted by a segment of white nationalists to appear less racist and extreme than “white nationalist” or “white supremacist;” the term “alt-right” isn’t used as widely now as it was when the census was conducted. While the labels they adopt may have changed, the accounts identified by the Alt-Right Census are still examples of the kinds of white supremacy that proliferates online; therefore, even though the data is a couple of years old, it is still useful for our purposes.
Once we had data from Twitter, Stormfront, and Reddit, we used two different types of computational analysis to determine what makes white supremacist speech unique and how it changes over time: text similarity and topic modeling. The first type of text-similarity analysis we used was text frequency-inverse document frequency (TF-IDF), which helps identify words that are unique to particular texts. Then, we used Shifterator,[74] a tool for comparing pairs of texts to identify their differences. We used dynamic topic modeling, which helps us to identify changes in the topics discussed over time. Together, these three approaches build on each other to help us understand both individual documents and larger sets. TD-IDF shows us what’s unique in an individual document; Shifterator reveals differences in pairs of documents. Topic modeling shows differences within larger sets of documents.
Text similarity: TF-IDF & Shifterator
TF-IDF
TF-IDF stands for “text frequency-inverse document frequency,” and it is a statistical measure of how unique a given word is to a set of documents. TF-IDF is calculated by counting how many times a word appears in a document and then taking the inverse of how often that word appears in the whole set of documents. For example, if we have two tweets, we treat each tweet as a document.
We used a second set of text similarity measures called entropy. Entropy in texts is a measure of how surprising or unexpected a particular word or phrase is given the other words and phrases in the documents. Shifterator uses word shift graphs to visualize the entropy of words in pairs of documents. These graphs make it easier to see which words make the documents different and how much they contribute to those differences. The example Shifterator graph at the left compares all the Stormfront comments posted in 2019 (left, purple) with all the Stormfront comments posted in 2020 (right, yellow). The graph makes it easy to see that, besides the coronavirus pandemic, conversations in 2020 touched on Black Americans, policing, and Trump more often than those in 2019 did. We used Shifterator charts to identify words that were unique when we compared platforms over time, as in this example, and between platforms (see Figure 11).
Topics and changes over time: Dynamic Topic Modeling
While word shift approaches like Shifterator are useful for comparing pairs of texts, we needed a way to compare more than two texts at a time. We used dynamic topic modeling to do these more complicated comparisons of texts over time and between platforms. Topic modeling, generally, is a computational approach to identifying clusters of documents that share a common theme that isn’t explicit. Dynamic topic modeling allows us to study the evolution of these implicit (topic modelers call them “latent”) themes over time by connecting topics at one time to topics at another. We can then see how the specific words associated with the general topic have changed. For instance, our model identified a “U.S. President” topic in which posts were discussing the president. During some time windows, that included discussions of President Obama, and during others, President Trump. Modeling the topic dynamically lets us see connections between the discussions of the two presidents and study how the language used to talk about the president changed when the man in office changed. We use this dynamic topic modeling approach because it is likely that topics follow one another—what people talk about at one time is related to what they talked about a short time before.
One challenge to note about topic modeling is that the algorithms return probabilistic values—the topic assignments are not certainties. Rather than saying, “this document belongs to this topic,” the algorithm returns a likelihood that a document or word belongs to a particular topic. Some words or topics may have similar likelihoods for more than one topic. The uncertainty that accompanies these probabilities should remind us that topic models are a useful but imprecise tool for understanding large collections of documents. It’s still important that we read texts to understand the distinctions and overlaps that topic modeling cannot detect.
One way to understand the similarity or difference of topics is to look at the words associated with them. To do so, we used word embeddings to represent the texts from each platform. Word embeddings are mathematical vector representations of words; words that are used in similar ways will have similar embeddings. Once learned, we can use these embeddings to compare how similar words are used on different platforms. For instance, how the word “Black” is used on Stormfront and Reddit is quite different. On Stormfront, “Black” is more similar to derogatory terms for Black people, and on Reddit, it’s more similar to other innocuous colors such as “green.” The different ways that words are used on different platforms and over time help us to understand what about a topic may have changed or why a word like “Jew” is more closely associated with white supremacy than with religion.
[73] Berger, Twitter Alt-Right Census, VOX-Pol, https://www.voxpol.eu/download/vox-pol_publication/AltRightTwitterCensus.pdf.
[74] Shifterator, “Latest,” https://shifterator.readthedocs.io/en/latest/.
Interactive Visualization: Topic Modeling
These interactive visualizations allow you to explore relationships between terms and topics and to see how topics related to one another on Stormfront and among the "alt-right" Twitter network. In each, the left side shows how similar topics are to one another (position) and how many documents they contain (circle size). The bar charts on the right size show how frequently terms appear in a selected topic (or the corpus if nothing is selected).
The visualizations show how we sorted pieces of text into topics based on the similarity of the words used, using a Latent Dirichlet Allocation (LDA) algorithm. The numbered "bubbles" represent the topics. The size of the bubbles is proportional to how many pieces of text the algorithm has sorted into that topic, and the distance between bubbles is proportional to their similarity: close or overlapping bubbles represent similar topics, while bubbles far apart represent topics that are different from each other.
The bar graph on the right of each shows the thirty most salient terms overall. By hovering the cursor over the bubbles, the bar graph will automatically update to show the most representative terms of the corresponding topic. By hovering the cursor over the words listed in the bar graph, the size of the bubbles will change to represent how frequently the corresponding topic uses that word. More advanced uses for these widgets can be found in the pyLDAvis documentation.
Topic Modeling: Alt-Right Twitter Census